Unsere vernetzte Welt verstehen
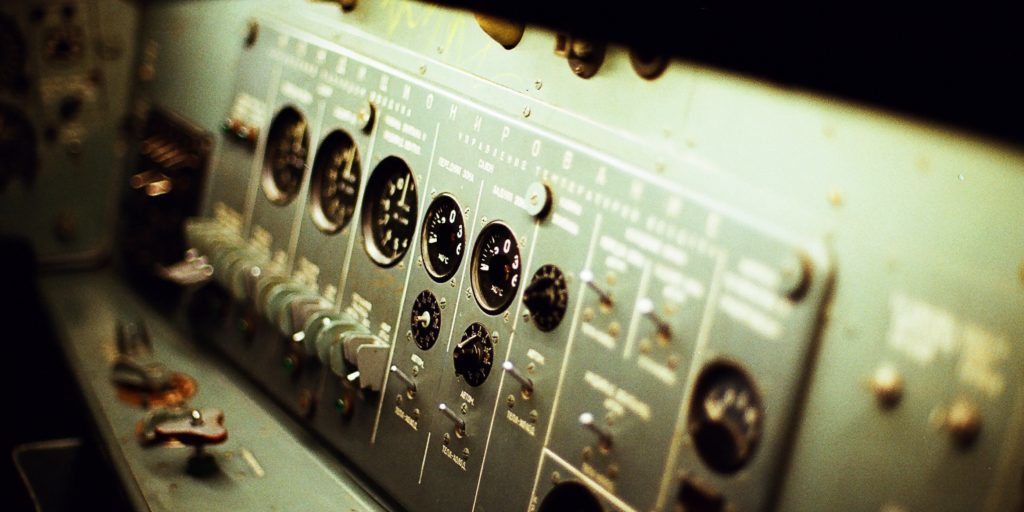
Qualitätskontrolle für Predictive Analysis
Immer mehr Entscheidungen werden mit Hilfe von Algorithmen getroffen. Eine Regulierung ist zwingend notwendig. Der assoziierte HIIG-Forscher Gert G. Wagner und Johannes Gerberding (Wissenschaftlicher Mitarbeiter in der Geschäftstelle des Sachverständigenrates für Verbraucherfragen: SVRV) geben in diesem Artikel eine Vorstellung davon, wie eine solche Regelung aussehen könnte.
The progress currently being made in the field of artificial intelligence is largely down to advances in the automatically generated prediction of human behaviour. What film should a streaming service recommend in order to keep subscribers coming back for more? Which job applicant will perform best in the vacant position? What are the chances of an insurance policy holder being involved in a traffic accident in the coming year? Or to suggest an example that is both compelling and disturbing: how probable is it that the nervous gentleman in the grey suit who is going through passport control at the airport is planning a terrorist attack?
Predictive Analytics: raising question on individual autonomy
The digital technology of predictive analytics is booming. And it raises difficult questions. What happens to the autonomy and self-determination of those subjected to such technology? Remember that it is not the actual person being analysed here but rather a person’s digitally constructed alter ego – a composite entity made up of isolated data points, resulting, for instance, from previous online activities, group-based statistical data aggregates and mathematical models. In a democratic society, shouldn’t we thus be giving priority to human judgement – despite all its well-known shortcomings, distortions and tendency towards discrimination? Shouldn’t we be imposing a general ban on digital prediction in certain social, economic or administrative contexts? Or is it enough to ensure that the programming routines used to generate predictions are made transparent? And that being the case, should they be made transparent for everyone or just for supervisory authorities? In order to answer these questions, we need to assess the value of trade secrets and determine the extent to which citizens and supervisory authorities should be allowed to inspect an algorithm’s inner workings and take action if required.
From predictive policing to people analytics
And those are just the most important aspects from the current perspective. Even a brief glance at the issues mentioned above shows how complex the deliberations among the relevant stakeholders will be if we set about tackling these regulatory questions. Against this backdrop, an overarching all-purpose “Algorithm Act” seems neither desirable nor realistic. In order to be fit for purpose, statutory regulation will have to take a more detailed, experimental and adaptive approach. The regulation of credit scoring agencies may serve as a starting point for regulating predictive analytics more generally. Because due to the onward march of predictive analytics, the way in which the probability value for a certain future event – in the case of credit scoring agencies: the probability of default – is ascertained to becoming a key issue in ever more aspects of our lives. It ranges from policing (where automated forecasting goes under the heading of “predictive policing”) to human resources management that makes use of increasingly sophisticated “people analytics”. The EU lawmaker has taken a first step toward regulating such phenomena by introducing the notion of “profiling”. Profiling as being defined in the General Data Protection Regulation means any form of automated processing of personal data to evaluate certain personal aspects relating to a natural person. Several instances of predictive analytics and scoring are covered by this definition.
The EU lawmaker should take the vague and non-binding requirement that “the controller should use appropriate mathematical or statistical procedures for the profiling” (Recital 71 General Data Protection Regulation) as the starting point for introducing a kind of digital product safety law for predictive analytics. It would be advisable, however, to separate such a digital product safety law from data protection law. Quality issues in predictive technologies are clearly still a problem even in situations where no personal data is involved and where data protection law is not therefore applicable. Assessments of creditworthiness are a concrete example of this. It makes no meaningful difference whether Ms X or Company Y gets a negative credit rating due to badly designed scoring technology.
Missing protection for victims of predictive analytics
The requirement for profiling to be based on “appropriate mathematical or statistical procedures” needs to be expanded upon and defined in greater detail. The said requirement surely rules out predictive technologies that are based on pure chance or on the forecaster’s non-rational intuition. It also means that it will be illegal to use technology which contains mistakes made by the statisticians during the design process. This is better than nothing, but it is less than it seems – because the “statistical procedures”-criterion itself does not oblige forecasters to ensure that their results meet any defined quality standards. Even if statistical methods are properly applied, the result can be scoring technology that is only marginally better than pure guesswork or even worse.
Furthermore, there is also a fairness aspect about the question concerning the quality of predictive analytics, as the validity and reliability of predictive technologies can vary in terms of how entire groups are evaluated. This is the case, for example, if women are more frequently rated as being “not creditworthy” than men even though there is no actual difference in the probability of loan repayment between the two groups, or even if women are in fact more creditworthy.
The uncertainties and distortions that can affect these predictions are not immediately visible. Anyone who reads a newspaper is familiar with election forecasts, but only a few specialists are aware of the different levels of uncertainty among them. When a profiling result, for example a credit score, is communicated, the uncertainty involved in its calculation is never mentioned. The purely abstract requirement of being based on “mathematical or statistical procedures” lends an air of pseudo-objectivity to the profiling results. Current legislation offers no protection here. This is where the lawmaker could step in.
Stricter than the status quo in data protection law
The experts in the specialist discipline of statistics and its newer offshoots (going by names such as data science) are obviously familiar with the lively debate on the various quality criteria for predictive technologies, including the discussion about what exactly these criteria tell us and where their respective strengths and weaknesses lie. Of particular relevance here are the criteria used in evaluating the quality of medical tests (“Is the patient infected with a specific pathogen?”) such as the false positive rate (“Not infected despite abnormal test results”) and the false negative rate (“Infected despite normal test results”). Lawmakers need to tap into this discussion. They could then exploit the fact that algorithms can be systematically tested in order to ascertain whether the results they generate are good enough in terms of predictive quality and fair enough in terms of group-related aspects.
If lawmakers and regulators get as far as tackling the concrete results of algorithms, there is a real chance of creating precisely tailored legislation that takes into account the particularities of the different areas in which predictive technology is applied. In certain areas, legislation of this kind will certainly be stricter than the status quo in data protection law. Aiming at substantial effectiveness, any regulation of algorithms will have to stipulate quality standards for results instead of merely requiring the technology to be based on mathematical or statistical procedures. Because as has already been shown, this approach leaves room for alarming errors and biases, thus generating results that can mark the affected persons with a permanent blemish like a scar. Better to avoid being scored at all than to be scored in a highly invalid and biased manner.
Where the scoring technology in question has no particular adverse impact or can easily be avoided by consumers (for example suggestions for music), there is no need for statutorily defined quality standards. And in contexts where the adverse effects of low-quality predictive scoring are mainly felt by the party using the predictions, market forces are strong enough to ensure that the users will either invest in improving the technology or abandon it altogether. But where the potential impact of an inaccurate prediction is significant and is mainly borne by the people whose lives and behaviour are being predicted, there is a need for higher standards than the purely formal criterion of whether the predictive technology is scientific. While credit scoring is the most obvious example here, another candidate for stricter quality standards of profiling is predictive analytics in the field of human resources, where artificial intelligence is often involved in determining the entire direction of a person’s future life. In short: it is absolutely essential that we hold a discussion about the specific social relevance and quality of digital algorithmic decision-making technologies in different areas of everyday and commercial life. Vague demands for an “Algorithm Act”, on the other hand, will not suffice.
Professor Gert G. Wagner is an economist and Research Associate at the Alexander von Humboldt Institute for Internet and Society in Berlin as well as Max Planck Fellow at the MPI for Human Development in Berlin; he is a member of the Advisory Council for Consumer Affairs (SVRV) and the German Academy of Science and Engineering (acatech); Johannes Gerberding is a lawyer and research consultant at the SVRV. This article is based on an article in German which appeared in “FAZ Einspruch” on 5 June 2019 (https://www.faz.net/einspruch)
Dieser Beitrag spiegelt die Meinung der Autorinnen und Autoren und weder notwendigerweise noch ausschließlich die Meinung des Institutes wider. Für mehr Informationen zu den Inhalten dieser Beiträge und den assoziierten Forschungsprojekten kontaktieren Sie bitte info@hiig.de
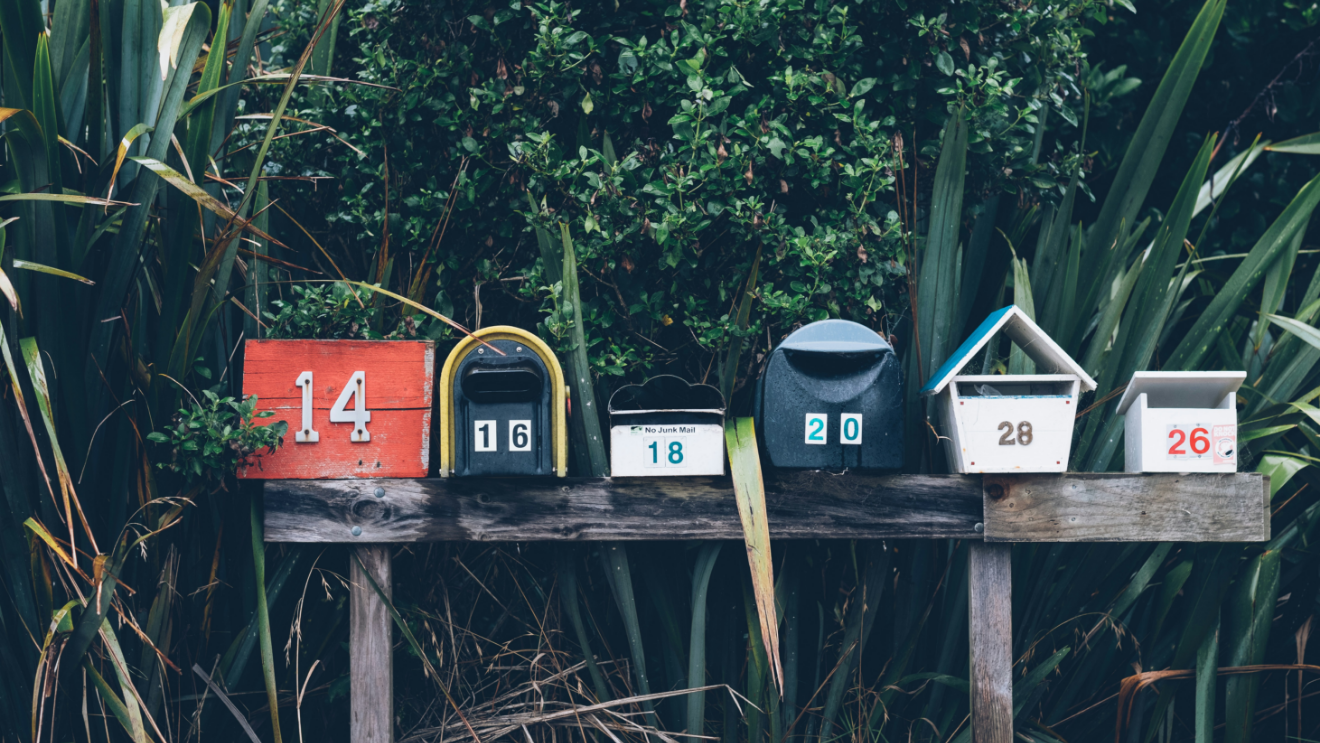
Jetzt anmelden und die neuesten Blogartikel einmal im Monat per Newsletter erhalten.
Forschungsthemen im Fokus
Raus aus dem Digitalisierungsstau: Data Governance bringt Städte und Gemeinden auf die digitale Überholspur
Der Data Governance Wegweiser unterstützt Verwaltungen, digitale Lösungen effektiv umzusetzen.
Netzecho: Reaktionen auf die Tagesschau in Einfacher Sprache
Seit 2024 gibt es die Tagesschau in Einfacher Sprache. Wie wird das neue Nachrichtenformat von Nutzer*innen im Internet diskutiert?
Chancen gegen Einsamkeit: Wie Pflegeeinrichtungen das Quartier vernetzen
Was hilft gegen Einsamkeit im Alter? Pflegeeinrichtungen schaffen neue Räume für Gemeinschaft und digitale Teilhabe.