Unsere vernetzte Welt verstehen
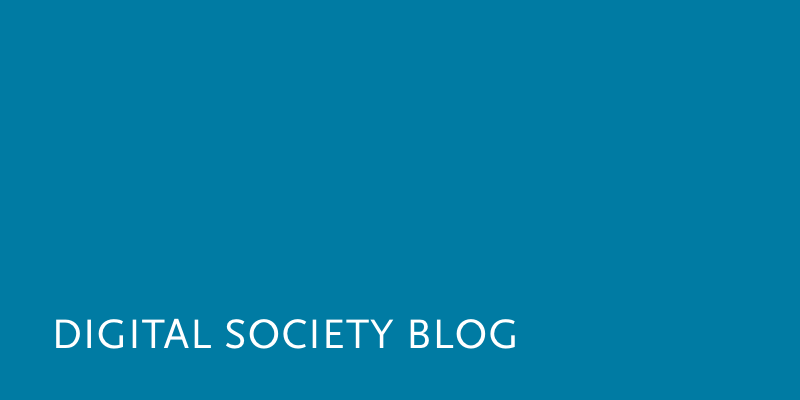
Profiling von Arbeitslosen
Seit 2014 nutzen polnische Jobcenter ein algorithmisches Entscheidungssystem, um Arbeitslose zu kategorisieren und verschiedene Unterstützungsangebote zu verteilen. In den konjunkturschwachen Zeiten nach der globalen Finanzkrise eingeführt, sollte das Profiling von Arbeitslosen die Effizienz der Jobcenter verbessern und die Unterstützung an individuelle Bedürfnisse anpassen. Allerdings erwies sich dieses neue Tool als Herausforderung für transparente Entscheidungsfindung und die Anwendung sozialer Rechte. Dieser Artikel diskutiert die Erkenntnisse aus dem Arbeitslosen-Profiling als Beispiel automatisierter Entscheidungen in der öffentlichen Verwaltung.
Process of categorization
Profiling mechanism is a scoring system aiming to divide unemployed people into three categories. The system is based on processing personal data, which is collected through a survey and an interview – a total of 24 data points. Each of them is assigned with specific volume of scores (0-8). Eight data points are collected during the registration at the job centre – for example, age, gender, disability, knowledge of foreign languages or duration of unemployment. Another 15 data points are gathered during the computer-based interview. The questions are constructed in a way that suggests that they are open-ended in character. But in reality, the scope of the answers is closed. For example, the question “What is the main obstacle for returning to the job market” has 22 predetermined answers. Based on the final score an algorithm decided which category should be given to the unemployed. The final calculation determines the scope of assistance which a person can apply for. According to statistics profile I covers 2% of the unemployed in Poland, profile II – 65%, and profile III – 33%.
More articles about algorithmic decisions and human rights
Social and legal implications
From the beginning, this profiling mechanism caused many confusions among clerks and unemployed people. Citizens applying for the assistance at job centres didn’t know the nature of this mechanism and its outcomes. In many cases assigned profiles were treated as blockades for receiving specific types of assistance. However the rules were constructed that it was difficult to change a person’s category. In practice, unemployed persons were trying to game the system in various different ways.
Polish Data Protection Authority, the Ombudsman, civil society organizations and trade unions were raising concerns over lack of specific safeguards for unemployed and imprecise scope of processed data. Now even the Polish government admits that there are some problems. The Ministry of Family, Labour and Social Policy recently informed that the government is planning to change the mechanism. However, no draft proposal was presented to the public yet. Meanwhile the Ombudsman referred profiling case to the Constitutional Court.
Further lessons
The profiling case raises few important lessons for introducing automated or semi-automated decision-making systems in public administration:
- The translation of the law into a code must be subject to democratic control. One of the biggest problems in the profiling case was an inaccuracy between the legal text and the IT systems functionalities (e.g. the scope of processed data or rules of changing the assigned profile). Policy makers should pay attention how system developers are translating legal provision into the code. Creating new automated-decision making systems in public administration could affect thousands of people in the standardize way and at the end lead to creation of new legal norms. This translation process should be subject to detailed supervision by the relevant institutions like courts, regulators or special parliamentary commission.
- People are using technologies in different ways. Initially, this profiling mechanism was designed to be only an advisory tool – it was a clerk who should make final decision about the profile. However research has shown that clerks in job centers apply differentiated strategies for using profiling mechanism. For many, the computer was a definitive decision-maker. For others, profiling was just a part of the broader diagnosis process. Another strategy was adjusting the profile to the expectations of the unemployed person. These examples show that in practice, the use of automated technologies depends on institutional issues, competencies and individual preferences. Designers’ intentions may be significantly different from the actual use of technology, and the level of automatization results not only from initial assumptions, but above all from the practice of users.
- Transparency and other safeguards are crucial. The process of profiling plays crucial role in shaping the unemployed situation. However the logic behind the profiling and the algorithm itself were treated as confidential information. As a result, the unemployed didn’t know how certain individual features or life circumstances affect his chance of being assigned to a given category. The questions which are asked during the interview with the unemployed became public only after an intervention from the Panoptykon Foundation. Scoring rules were published after the court’s judgment. This case shows that a person subjected to automated decisions should have a legal right to obtain detailed information about all aspects of this process that might affect her situation (including the logic behind it, what data was used and with what result etc.), as well as to be offered human intervention and explanation of the final result.
- Courts and human rights institutions must learn how to work with algorithms. Profiling the unemployed was subject of intervention from the Ombudsman, Data Protection Authority and courts. The scope and level of these interventions vary. However, this examples show that the technological layer is a problem in assessing the consequences for human rights. In such situations, the institutions should cooperate and exchange their experiences (e.g. DPA in the technical aspects of data processing, and the Ombudsman in the context of discrimination). We also have to consider whether algorithms used in the process of decision-making by public administration shouldn’t be subjected to prior approval of independent bodies capable of evaluating human rights-related risks.
- Not all decisions should be automatized. In the pursuit of increasing the efficiency of public administration through automatization, one should ask which processes can be automated and which should not. Elements that should be taken into account should be the scope of discretion, rule of law principles, potentially negative effects on citizens and the consequences for human rights.
Jędrzej Niklas erhielt seinen PhD im Bereich International Law. Aktuell arbeitet er am Department of Media and Communications an der London School of Economics (LSE) und ist Fellow beim Centre for Internet & Human Rights an der Europa-Universität Viadrina. Seine Forschung konzentriert sich auf die Beziehung von Menschenrechten und datengetriebenen Technologien. Dieser Text basiert auf der Publikation Profiling the Unemployed in Poland: Social And Political Implications Of Algorithmic Decision Making.
Der Artikel ist Teil eines Dossiers über algorithmische Entscheidungen und Menschenrechte. Sie möchten selbst einen Artikel im Rahmen dieser Serie veröffentlichen? Dann senden Sie uns eine Email mit Ihrem Themenvorschlag.
Dieser Beitrag spiegelt die Meinung der Autorinnen und Autoren und weder notwendigerweise noch ausschließlich die Meinung des Institutes wider. Für mehr Informationen zu den Inhalten dieser Beiträge und den assoziierten Forschungsprojekten kontaktieren Sie bitte info@hiig.de
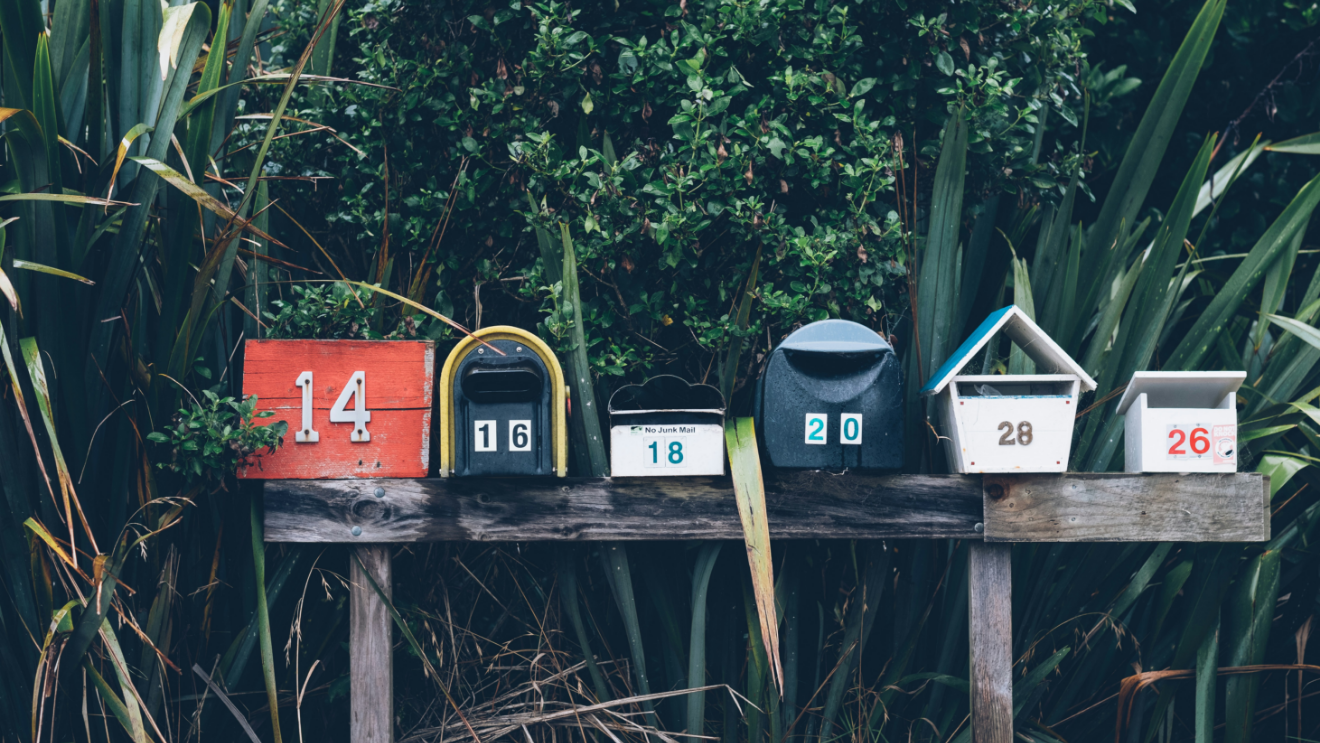
Jetzt anmelden und die neuesten Blogartikel einmal im Monat per Newsletter erhalten.
Plattform Governance
Zwischen Vision und Realität: Diskurse über nachhaltige KI in Deutschland
Der Artikel untersucht die Rolle von KI im Klimawandel. In Deutschland wächst die Besorgnis über ihre ökologischen Auswirkungen. Kann KI wirklich helfen?
Ein Schritt vor, zwei zurück: Warum Künstliche Intelligenz derzeit vor allem die Vergangenheit vorhersagt
KI gilt als Zukunftstechnologie, stützt sich selbst aber meist auf historische Daten. Reproduziert sie dadurch soziale Ungleichheiten und Verzerrungen?
Zwischen Zeitersparnis und Zusatzaufwand: Generative KI in der Arbeitswelt
Generative KI am Arbeitsplatz steigert die Produktivität, doch die Erfahrungen sind gemischt. Dieser Beitrag beleuchtet die paradoxen Effekte von Chatbots.