Unsere vernetzte Welt verstehen
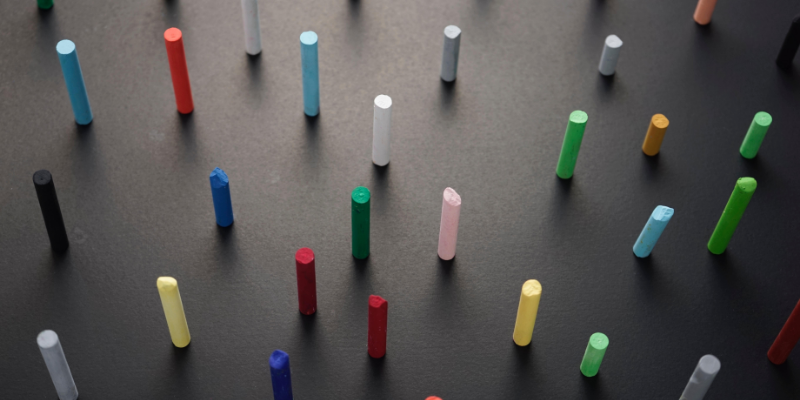
Explaining AI – Wie erklärt man das Unerklärliche?
Automatisierte Entscheidungsfindungssysteme (ADM) sind in unserem Alltag allgegenwärtig geworden und komplex zu verstehen. Sollte es uns also überhaupt interessieren, wie KI-gestützte Entscheidungen getroffen werden? Auf jeden Fall, denn der Einsatz dieser Systeme birgt sowohl auf individueller als auch auf gesellschaftlicher Ebene Risiken – wie z. B. fortbestehende Stereotypen oder falsche Ergebnisse aufgrund falscher Dateneingabe. Diese Risiken sind nicht neu und müssen diskutiert werden. Und dennoch gibt es deutliche Hinweise darauf, dass Menschen, die mit automatisierten Entscheidungen arbeiten, in fast 100 % der Fälle dazu neigen, den Systemen zu folgen. Wie können wir also Menschen befähigen, mit KI zu denken, sie zu hinterfragen oder herauszufordern, anstatt blind auf ihre Richtigkeit zu vertrauen? Die Lösung sind sinnvolle Erklärungen für Laien durch und über das System und sein Entscheidungsverfahren. Aber wie? Dieser Blogartikel zeigt, welche Kriterien diese Erklärungen erfüllen sollten und wie sie die Kriterien der Allgemeinen Datenschutz-Grundverordnung (DSGVO) erfüllen können.
Explainable AI – a possible solution
Explanations of how automated decision making (ADM) systems make decisions (explainable AI, or XAI) can be considered a promising way to mitigate their negative effects. Explanations of an ADM system can empower users to legally appeal a decision, challenge developers to be aware of the negative side effects, and increase the overall legitimacy of the decision. These effects all sound very promising, but what exactly has to be explained to whom, and in which way, to best reach these goals?
The legal approach towards a good explanation
To find an answer to this complex question, one could start looking at what the law says about ADM systems. The term “meaningful information about the logic involved”, found in the GDPR, could be seen as the legal codification of XAI within the EU. Although the GDPR is among the world’s most analysed privacy regulations, there is no concrete understanding on what type of information developers have to provide (and at which time and to what type of user).
Only some parts can be understood from a legal perspective alone: First, the explanation has to enable the user to appeal the decision. Second, the user needs to actually gain knowledge through the explanation. Third, the power of the ADM developer and of the user has to be balanced through the explanation. Last but not least, the GDPR focuses on individual rather than collective rights or in other words: an individual without any technical expertise must be able to understand the decision.
Interdisciplinary approach: Tech and Design
Since legal methods alone do not lead to a complete answer, an interdisciplinary approach seemed a promising way to better understand the legal requirements on explainable AI. A suggestion of what such an approach could look like is made by the interdisciplinary XIA report of the Ethics of Digitisation project. It combines views of legal, technical and design experts to answer the overarching question behind the legal requirements. What is a good explanation? We started with defining three questions towards a good explanation: Who needs to understand what in a given scenario? What can be explained about the system in use? And what should explanations look like in order to be meaningful to the user?
Who needs to know what?
What a good explanation looks like highly depends on the target group. For instance: In a clinic setting, a radiologists might need to know more about the general functioning of the model (global explanation) while a patient would need an explanation on the result of a single decision (local explanation).
Besides this expert (radiologist) and lay (patient) users, another target group of an explanation are public or community advocates. The advocate groups support individuals confronted with an automated decision. Their interest will be more in understanding the models and their limitations as a whole (global), instead of only focussing on the result of one individual decision (local). The importance of the advocates group is already understood in other political contexts in society, such as inclusive design for AI Systems, i.e., that design teams need more people of colour and women to avoid problems of bias and discrimination. They should also play a bigger role in the field of explainable AI.
The Design – What should explanations look like?
The type of visualisation also depends on the contexts, point in time, and, among many other factors, the target group. One answer to this question which could fit all types of explanations does not exist. Therefore, we propose to introduce a participatory process of designing the explanation into the development process of the ADM system. The advocates group should be part of this process representing the lay users. This might lead to an explanation to be “meaningful” to the user and compliant with the GDPR.
The technical view – What can be explained about the system in use?
A solution to provide an explanation might be post-hoc interpretations. They are delivered after the decision was made (post-hoc). An example is a saliency map, commonly used to analyse deep neural networks. These maps highlight the parts of the input (image, text, etc.) that are deemed most important to the model prediction. However, they do not prevail in the actual functioning of the model. Therefore, we do not conceive them to be able to empower the user to appeal a decision.
We rather propose making the underlying rationale, design and development process transparent and document the input data. This may require obligations to document the processes of data gathering and preparation including annotation or labelling. The latter can be achieved through datasheets. The method selection for the main model as well as the extent of testing and deployment should also be documented. This could be “the logic involved” from a technical perspective.
Another major issue of explainable AI are the so-called black box models. These are models which are perceived as non interpretable. However, such systems tend to come with a very high performance. Therefore, we propose to weigh up the benefits of high performance with the risks of low explainability. From a technical perspective, it might be useful to work with such a risk based approach, although this might contradict with the legal requirement of the GDPR to always provide an explanation.
Bringing the views together
As shown in this article as well as the report, law, design, and technology have a different, in some points even contradicting perspective on what “meaningful information about the logic involved” are. Although we did not find the one definition for these terms, we found some common grounds: The explanation should be developed and designed in a process involving representation of the user. The minimum requirement is documentation of the input data as well as architectural choices. However, it is unlikely that only documenting this process enables the user to appeal an automated decision. Therefore, other types of explanations have to be found in the participatory process in order to be compliant with the GDPR.
I would like to thank Hadi Ashgari and Matthias C. Kettemann, both also authors of the clinic report, for their thoughts and suggestions for this blogpost.
Dieser Beitrag spiegelt die Meinung der Autorinnen und Autoren und weder notwendigerweise noch ausschließlich die Meinung des Institutes wider. Für mehr Informationen zu den Inhalten dieser Beiträge und den assoziierten Forschungsprojekten kontaktieren Sie bitte info@hiig.de
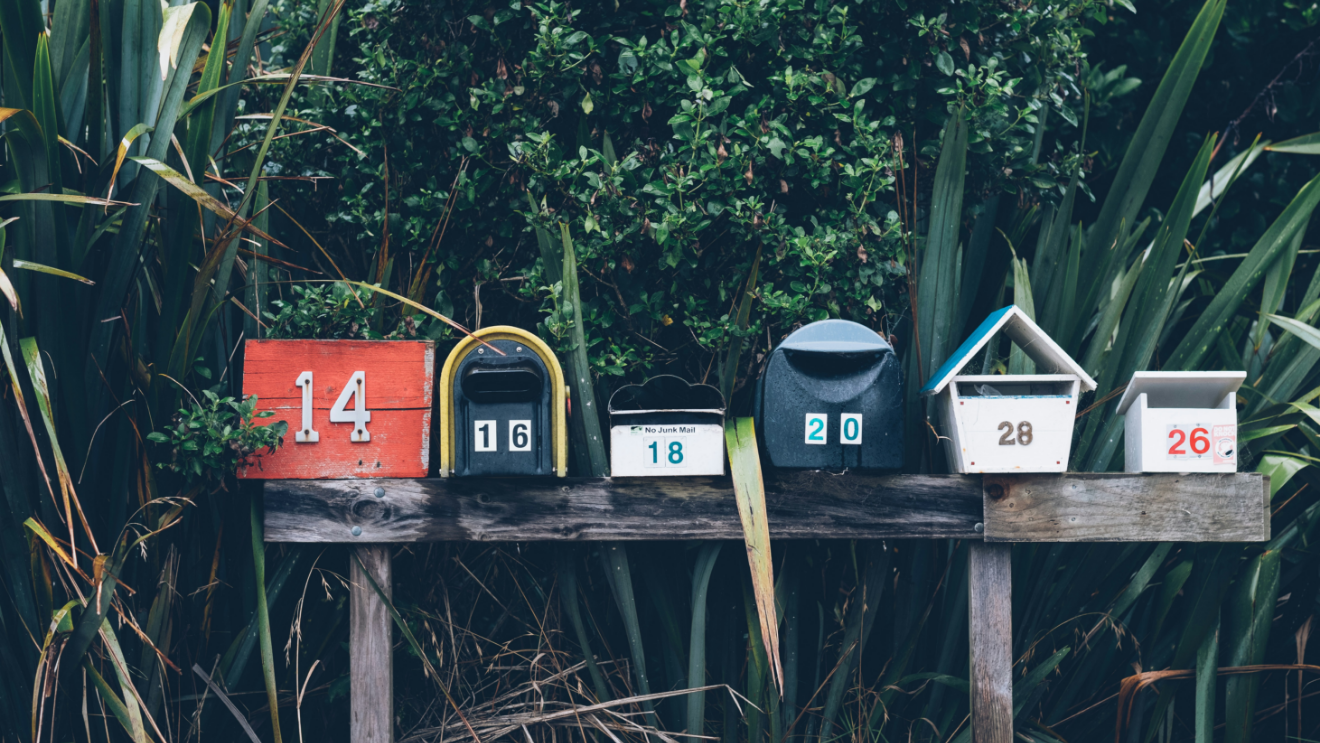
Jetzt anmelden und die neuesten Blogartikel einmal im Monat per Newsletter erhalten.
Künstliche Intelligenz und Gesellschaft
Raus aus dem Digitalisierungsstau: Data Governance bringt Städte und Gemeinden auf die digitale Überholspur
Der Data Governance Wegweiser unterstützt Verwaltungen, digitale Lösungen effektiv umzusetzen.
Netzecho: Reaktionen auf die Tagesschau in Einfacher Sprache
Seit 2024 gibt es die Tagesschau in Einfacher Sprache. Wie wird das neue Nachrichtenformat von Nutzer*innen im Internet diskutiert?
Chancen gegen Einsamkeit: Wie Pflegeeinrichtungen das Quartier vernetzen
Was hilft gegen Einsamkeit im Alter? Pflegeeinrichtungen schaffen neue Räume für Gemeinschaft und digitale Teilhabe.