Unsere vernetzte Welt verstehen
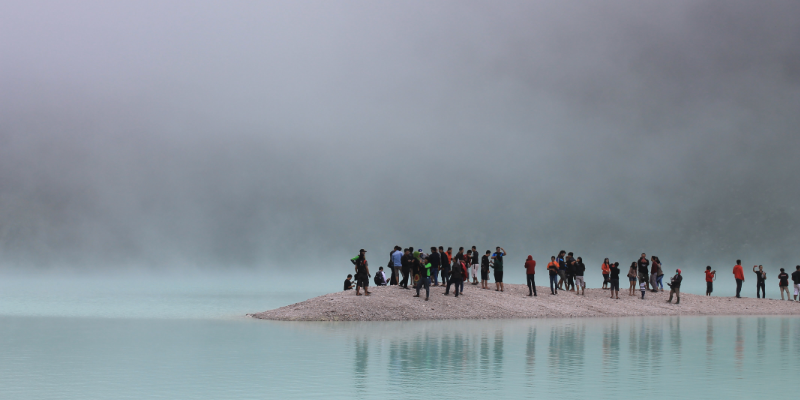
Warum explainable AI eine gesellschaftliche Perspektive braucht
Hast Du dich schon einmal gefragt, wie die automatischen Vervollständigungen deiner Suchanfragen in einer Suchmaschine zustande kommen? Zum Beispiel, als Dir vorgeschlagen wurde, zu suchen, wie es sich anfühlt, Sodbrennen zu haben, während Deine beabsichtigte Suche damit scheinbar überhaupt nichts zu tun zu hatte. Noch gibt es keinen Standard dafür, solche automatisierten Entscheidungen zu erklären. Darüber hinaus konzentrieren sich heutige Explainable AI (XAI) Frameworks stark auf individuelle Interessen, während eine gesellschaftliche Perspektive zu kurz kommt. Dieser Artikel führt in die zielgruppenspezifische Kommunikation in XAI ein und stellt die Figur des public Advocates vor, als eine Möglichkeit kollektive Interessen in XAI Frameworks einzubetten.
Der Artikel basiert auf den Überlegungen von Dr. Theresa Züger, Dr. Hadi Asghari, Johannes Baeck und Judith Faßbender während der XAI Clinic im Herbst 2021.
Missing or insufficient explainability for lay people and society
Have you ever asked yourself what the basis of your search engine autocompletions is? For example at that time when you typed “how does” and your search engine suggested “how does…it feel to die”, “how does…it feel to love”, “how does…it feel to have heartburn” but you actually wanted to continue typing “how does… a2 relate to b2 in Pythagoras’ theorem”. If explanations for automated decisions were a standard, you would have been able to get an explanation of the inner workings of that search engine fairly easily. Due to a mixture of technical feasibility, communicational challenges and strategic avoidance, such a standard does not exist yet. Whilst a number of major providers and deployers of AI-models have published takes on Explainable AI (XAI) – most prominently IBM, Google and Facebook – none of these efforts offer effective explanations for a lay audience. In some cases, lay people are simply not the target group, in others, the explanations are insufficient. Moreover, collective interests are not taken into account sufficiently when it comes to how to explain automated decisions; the focus lies predominantly on individual or private interests.
This article will focus on how explanations for automated decisions need to differ in regards to the audience that is being addressed – in other words, on target group specific communication of automated decisions. In light of the neglected societal perspective, I will introduce the figure of the public advocate as a possibility to include collective interests in XAI frameworks.
Technical elements of AI-systems to explain
The technological complexity of AI-systems makes the traceability of automated decisions difficult. This is due to models with multiple layers, nonlinearities and untidy, large data sets, amongst other reasons. As a reaction to this problem, there have been increasing efforts to develop so-called white-box algorithms or to use more simple model architectures which produce traceable decisions, such as decision trees.
But even if each element of an AI-system is explainable, a complete explanation for an automated decision would consist of a fairly large number of elements. To give an idea of these elements, let me share a dry yet helpful overview of possible elements (based on Liao et al. (2020)):
(1.) The global model which refers to the functionalities of the system that has been trained, this includes which training data has been used, and which architecture (i.e. a convolutional neural network, linear regression, etc.). Global means that the functionality of the system is not case-specific (2.) The local decision, which concerns a decision in a specific case. (3.) The input data which refers to the specific data a local decision is made on. (4.) The output refers to the format and the utilisation of the output the system gives (5.) A counterfactual explanation, which shows how different the input would have to be in order to get a different output; such as (6.) the performance of the system.
The challenge of target group specific communication
If what you’ve read up to now has either bored or overwhelmed you, it could either mean that you are not the target group for this blog post or that I have missed the sweet spot between what you, as part of my target group, knew already and what you expect from this article. Target group specific communication and hitting that sweet spot is a struggle when explaining automated decisions as well.
To give you a schematic, but better, explanation, here are the elements listed above, applied to the search engine example from the beginning of this blog post:
- The global model in this case is the trained model which produces the autocomplete suggestions, the training data is most probably previous inputs by other users, what they were searching and their entire search history.
- The input was what you typed in combination with your search history and other information the search engine provider has on you.
- The output is the autocomplete suggestion.
- The local decision is the suggestions you’ve been given, based on your input.
- A counterfactual could involve seeing what suggestions you would get when typing the exact same words, but taking parts of your search history out of the equation or changing another parameter of the input data.
- The performance of the system would be based on how many people do actually want to find out how it feels to die etc., as opposed to how Pythagoras’ theorem works.
The performance, for example, would probably not be interesting for the average lay person, which is different e.g. for the developer: People in different positions have different needs, expectations, and previous knowledge concerning explanations, and so therefore the type of presentation needs to differ for each target group.
Who asked?
The standard target groups for explanations of automated decisions – which are not catered to in the same manner – are the developer, the domain expert and the affected party.
The developers either build new AI-models or further develop pre-existing AI-models. This group basically needs to understand each element of the system, with a specific focus on the working of the global model and data representation – to be able to improve and verify the system in an accountable manner. Such explanations have to be available for developers throughout the whole process of development, employment and maintenance of the system.
The domain expert is typically an employee of an organisation which uses AI-systems. This could be a medical doctor assisted by an AI-system when making a diagnosis or a content moderator on a social media platform who checks automatically flagged content. This person is assisted in their decision-making with suggestions from an AI-system, as a so-called “human in the loop”. Domain experts need to adapt to working with the system and need to develop an awareness of risks, of misleading or false predictions as well as the limitations. Therefore they do not only need explanations of local decisions (e.g. why did the system flag this content as being inappropriate), but importantly a thorough training on how the global system works (e.g what data the system was trained on, does the system look for specific words or objects). Such a training needs to take place in connection to the specific use context.
The affected party is, as the name suggests, the person (or other entity) that an automated decision has an effect on. Their needs range from knowing if an AI-system was involved in a decision, to understanding an automated decision in respect to making informed decisions or to practice self-advocacy and challenge specific decisions or the use of an AI-system altogether. Affected parties primarily need an explanation on the elements of the system which are connected to their case (local decision). Counterfactual explanations can also be meaningful, as they would enable affected people to see what factors would need to change (in their input data) to produce a different result (the output).
A 4th target group: the public advocate
We propose considering a fourth target group: the public advocate.
The public advocate describes a person or an organisation which takes care of the concerns of the general public or a group with special interests. The general North Star of all public advocate activities has to be to move closer to equality in our understanding of this target group. A public advocate might be an NGO/NPO, dealing with societal questions connected to the use of AI-systems generally – such as e.g. Access Now, Algorithmwatch or Tactical Tech – or an NGO/NPO with a focus on specific groups or domains e.g. the Ärztekammer or organisations supporting people who are affected by discrimination.
The concern of public advocates is on one hand lobbying and advocating for the public interests or special needs – this may be in deliberative processes in media, in court, in policy-making or in collaboration with providers of AI-systems. On the other hand, such organisations are well-qualified to educate others on AI-systems, tailored to the needs of their respective community. This might be the Ärztekammer (professional representation of medical doctors in Germany) providing radiologists (domain experts) with training and background information on the possibilities, risks and limits of e.g. image recognitions of a lesion in the brain.
To facilitate such support, these groups need access to general information on the AI-system – to the global functioning of the model, input, and output. Further explanations of individual cases and the impact on individuals is crucial for this group, especially when their advocacy focuses on specific societal groups or use cases.
Why is a collective perspective in explainable AI important?
The field of XAI is not free of power imbalances. Interests of different actors interfere with one another. Against this backdrop, the need of having a public advocate becomes more clear: None of the traditional target groups are intrinsically concerned with collective interests and consequences. But a collective focus is important, especially with regards to seemingly low-impact decisions e.g. which content is suggested to you on platforms or search engines. These automated decisions may count as low-impact in isolation, but can become problematic with scaling the number of users and/or decisions – e.g. when Facebook’s recommendation tool contributed to the growth of extremist groups. Whilst high impact decisions for individuals – such as the often cited loan-lending case – are highlighted in XAI frameworks, “low-impact” decisions are much more in the shadows, but viewing them from a societal, collective perspective sheds some light on their importance. The content that is suitable for an explanation from this perspective is different, and it can be formulated by considering the target group of the public advocate.
Besides the representation of collective needs, public advocates can take over important tasks in the field of explainable AI. Training sessions on how specific AI-systems work should be given by an entity that does not develop or employ such systems themself and therefore does not have obvious conflicting private interests – which rules out commercial actors and governmental organisations. The public advocate can function as a consultant to the developing teams if they are included early enough in the development process and if there is a true interest in giving effective explanations.
Last but not least, public advocates have more leverage than a singular affected person when lobbying for a collective. In comparison to the layperson, the organisations we have in mind have more technical expertise and ability to understand how the system works which increases their bargaining power further. Ideally, the work of the public advocates reduces the risk of ineffective explanations which are more a legal response than actual attempts to explain – see Facebook’s take on explaining third party advertisements.
For all points mentioned above – automated decisions which become critical when viewed on a collective scale, the need to have a publicly minded entity to educate on AI-systems and the benefits of joining forces with different affected parties – there needs to be a ‘public advocate’ in XAI frameworks. Not only to consequently include the societal and collective dimension when offering affected users explanations but to make collective interests visible and explicit for the development of explainable AI in the first place.
Further reads
Carvalho, D. V., Pereira, E. M., & Cardoso, J. S. (2019). Machine learning interpretability: A survey on methods and metrics. Electronics, 8(8), 832.
Liao, Q. V., Gruen, D., & Miller, S. (2020). Questioning the AI: informing design practices for explainable AI user experiences. In Proceedings of the 2020 CHI Conference on Human Factors in Computing Systems (pp. 1-15).
Ribera, M., & Lapedriza, A. (2019). Can we do better explanations? A proposal of user-centered explainable AI. In IUI Workshops (Vol. 2327, p. 38).
Rohlfing, K. J., Cimiano, P., Scharlau, I., Matzner, T., Buhl, H. M., Buschmeier, H., … & Wrede, B. (2020). Explanation as a social practice: Toward a conceptual framework for the social design of AI systems. IEEE Transactions on Cognitive and Developmental Systems, 13(3), 717-728.Miller, T. (2019). Explanation in artificial intelligence: Insights from the social sciences. Artificial intelligence, 267, 1-38.
Dieser Beitrag spiegelt die Meinung der Autorinnen und Autoren und weder notwendigerweise noch ausschließlich die Meinung des Institutes wider. Für mehr Informationen zu den Inhalten dieser Beiträge und den assoziierten Forschungsprojekten kontaktieren Sie bitte info@hiig.de
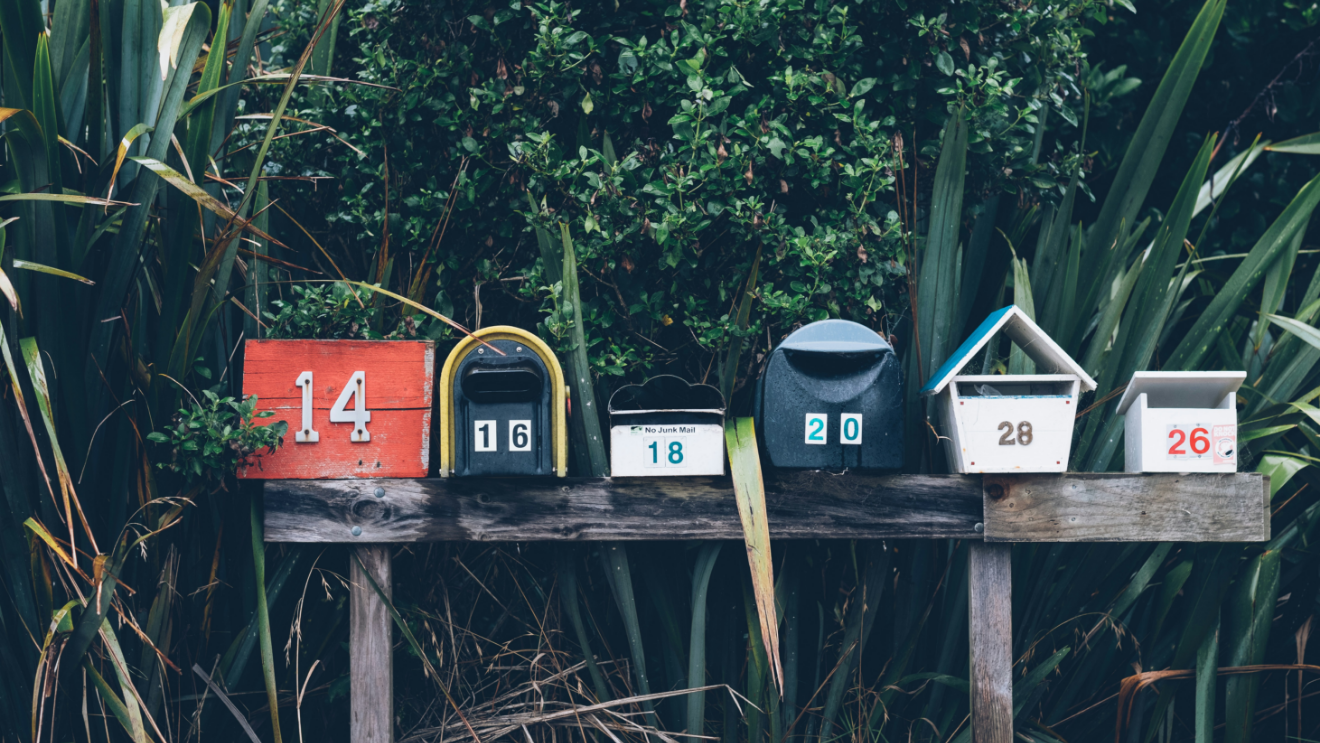
Jetzt anmelden und die neuesten Blogartikel einmal im Monat per Newsletter erhalten.
Künstliche Intelligenz und Gesellschaft
Die Macht der Plattformen: Die Zukunft der Regulierung nach der Europawahl
Rückblickend auf die Europawahlen 2024 zieht dieser Beitrag Bilanz über die Auswirkungen des Gesetzes über digitale Dienste auf die Macht der Plattformen.
KI unter Aufsicht: Brauchen wir ‘Humans in the Loop’ in Automatisierungsprozessen?
Automatisierte Entscheidungen haben Vorteile, sind aber auch fehleranfällig. Ein Human in the Loop könnte helfen. Aber garantiert er bessere Ergebnisse?
Die Vielfalt von digitalen B2B-Plattformen
Dieser Blogbeitrag untersucht die Vielfalt digitaler Business-to-Business-Plattformen und kategorisiert sie nach Governance-Stilen und strategischen Zielen.