What to explain when explaining is difficult. An interdisciplinary primer on XAI and meaningful information in automated decision-making
Author: | Asghari, H., Birner, N., Burchardt, A., Dicks, D., Fassbender, J., Feldhus, N., Hewett, F., Hofmann, V., Kettemann, M. C., Schulz, W., Simon, J., Stolberg-Larsen, J., & Züger, T. |
Published in: | HIIG Impact Publication Series |
Year: | 2022 |
Type: | Other publications |
DOI: | 10.5281/zenodo.6375784 |
Explanations of how automated decision making (ADM) systems make decisions (explainable AI, or XAI) can be considered a promising way to mitigate their negative effects. The EU GDPR provides a legal framework for explaining ADM systems. “Meaningful information about the logic involved” has to be provided. Nonetheless, neither the text of the GDPR itself nor the commentaries on the GDPR provide details on what this precisely is. This report approaches these terms from a legal, technical and design perspective.Legally, the explanation has to enable the user to appeal the decision made by the ADM system and balance the power of the ADM developer with those of the user. “The logic” can be understood as “the structure and sequence of the data processing”. The GDPR focuses on individual rather than collective rights. Therefore, we recommend putting the individual at the centre of the explanation in a first step in order to comply with the GDPR.From a technical perspective, the term “logic involved” is – at best – misleading. ADM systems are complex and dynamic socio-technical ecosystems. Understanding “the logic” of such diverse systems requires action from different actors and at numerous stages from conception to deployment. Transparency at the input level is a core requirement for mitigating potential bias, as post-hoc interpretations are widely perceived as being too problematic to tackle the root cause. The focus should therefore shift to making the underlying rationale, design and development process transparent—documenting the input data as part of the “logic involved”. The explanation of an ADM system should also be part of the development process from the very beginning.When it comes to the target group of an explanation, public or community advocates should play a bigger role. These advocate groups support individuals confronted with an automated decision. Their interest will be more in understanding the models and their limitations as a whole instead of only focussing on the result of one individual decision.
Visit publication |
Download Publication |
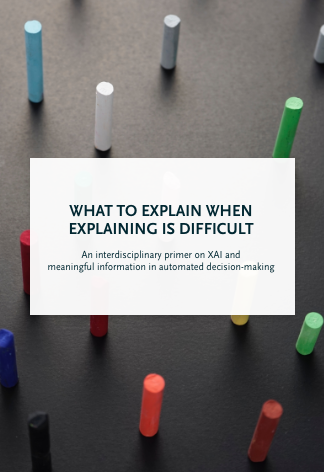
Connected HIIG researchers
Vincent Hofmann
Jakob Stolberg-Larsen
Hadi Asghari, Dr.
Freya Hewett
Judith Faßbender
Nadine Birner
Daniela Dicks
Matthias C. Kettemann, Prof. Dr. LL.M. (Harvard)
Theresa Züger, Dr.
Wolfgang Schulz, Prof. Dr.
- Open Access