Making sense of our connected world
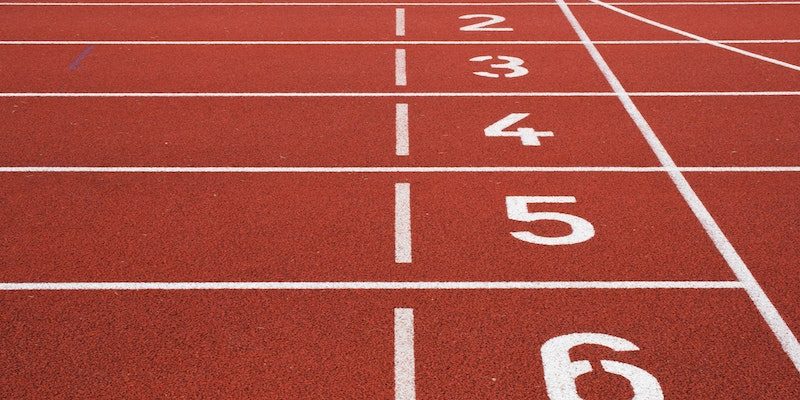
Opening match: the battle for inclusion in algorithmic systems
Team civil society and team industry go head-to-head on conditions and rules for inclusive design
How can the increasing automation of infrastructures be made more inclusive and sustainable and be brought into accordance with human rights? The AI & Society Lab pursues this core issue by facilitating exchange between academia, industry and civil society while experimenting with different formats and approaches. As one of its initial ventures, it hosted a series of roundtables in cooperation with the Representation of the European Commission in Germany to work on the implementation and operationalisation of the commission’s White Paper on AI.
To extend and sustain the societal debate on inclusive AI, the topic of our third roundtable, we challenged two stakeholder groups to a ping pong match, the world’s fastest return sport – but digitally, and with the AI & Society Lab hitting the first serve. Playing for team civil society was Lajla Fetic, scientist and co-author of the Algo.Rules, a practical guide for the design of algorithmic systems. Facing her on the other side of the net was Finn Grotheer, AI business development fellow at Merantix, a Berlin-based AI venture studio. On your marks, get set, go!
What AI topic won’t let you sleep at night?
Finn: In particular, so-called GANs (general adversarial networks) are a major societal challenge. They can artificially generate videos and soundtracks that are not recognisable as fakes. In light of our social media culture and its influence on society and politics, we can only hint at their effect.
Lajla: The hype about AI does not give me nightmares. What I ponder are the questions behind it: how can all people benefit equally from technology? How can marginalised groups find a hearing in the design of AI? If women, people with disabilities, people with migration experiences or without a university degree can participate equally in debates and in the development of AI, I will sleep even better.
What AI topic has not yet been sufficiently discussed?
Finn: Competitiveness. It is neither sexy nor does it spark enthusiasm. But we will only be able to implement our ethical standards if we – Germany and Europe – take the lead in developing, testing and scaling the best AI applications. Otherwise, we run the risk of repeating the experiences we are currently having with the American internet giants.
Lajla: The “why” question is too seldom asked in this highly charged debate. For what purpose are we developing algorithmic systems and at what cost? As a rule of thumb: More is not always better. Algorithmic systems offer a lot of (unused) potential. And we have to discuss the conditions under which the latest developments are coming about. Training complex machine learning systems takes a lot of energy. Tools that make invisible CO2 costs visible are a good first step in talking about common good-oriented goals of technology design.
What should AI be able to do today rather than tomorrow?
Finn: What AI can do isn’t necessarily the bottleneck. Much of its potential is simply still untapped. In healthcare, for example, people around the world still die of treatable diseases because they have no access to diagnostics and treatment. We underestimate how much we could already achieve today with a worldwide penetration of tested AI applications.
Lajla: It is important to me that, today rather than tomorrow, we develop solutions for how we can use AI as a tool in a meaningful way. This requires an understanding of the possibilities and limitations and how the interaction between man and machine really works.
“We can learn to deal with bias in mind and code through critical reflection”.
Lajla, developing inclusive and non-discriminatory AI – is that even possible?
The inclusive design of AI is a big task for the next years – there will never be discrimination-free AI. How could there be? Are we humans free of prejudice? But we can learn to deal with bias in our heads and code by critical reflection. Rules for the design of algorithmic systems help us to do so. For example, it is only through good documentation of the processed data and evaluation criteria we can determine whether a certain group of people does worse due to the use of technology.
Finn: Definitely: Design can be conducted inclusively, and that is an undisputed priority. However, the fact that AI systems will never be free of all discrimination cannot only be attributed to human biases during the design process. Self-learning systems are trained on data sets that, in the first step, were usually not subject to conscious human selection. Instead, they are a product of their environment. For example: available images or speech samples. This is precisely where the challenge lies. Therefore, an important measure is to institutionalise mechanisms that flag up biases noticed in production.
Laijla: To ensure that AI does not reproduce and scale existing prejudices, those developing and implementing AI must take responsibility from the very beginning. The training data sets are compiled, curated and labelled in advance. During this process, many things – man-made – can go wrong. An experiment by Kate Crawford showed how the well-known ImageNet data set (a set with more than 14 million images that is the basis for many object recognition systems) produced misogynistic results – due to bad labels. In order to select good data sets and avoid possible (gender) data gaps, we need measures to address the garbage-in-garbage-out phenomenon at an early stage. A first step would be to assemble more diverse and sensitised developer teams. Another one would be to introduce quality standards for data sets that also pay attention to representation, such as the Data Nutrition Label from Harvard University and MIT Media Labs.
Finn: There is no disagreement here. Developer teams are happy to take on the responsibility. It’s not like there aren’t any quality standards; the relevance of training data is especially well known. All serious companies work daily on the most representative and unbiased data sets possible. Diversity in teams is a very helpful maxim on an individual level, but in the AI industry as a whole, teams can of course only reflect the degree of diversity that comes from universities.
Lajla: It’s not that easy with quality standards. The documentation – for instance on training datasets for ML models – is not comprehensive in many cases. Often start-ups and smaller companies cannot afford the expense of adequate documentation and ethical due diligence. Therefore, mandatory minimum standards help us to firmly anchor what is socially desirable in corporate practice. In terms of diversity, I agree with you: we need to start much earlier, increase the proportion of female students in STEM subjects, break down social barriers and take the shortage of skilled workers seriously.
Finn: When it comes to minimum standards, I wonder whether a law can reflect the complexity, diversity and pace of the AI industry. We work a lot with industrial clients – manufacturing, e-commerce, synthetic biology – and use their data to develop customised systems, for instance for improving production or quality control. Each industry has its own characteristics and not every B2B use case raises ethical questions. I would be interested to hear what legal minimum standards would look like specifically and under which circumstances they would apply.
AI systems could potentially make discrimination visible, but they are mostly used for reasons of efficiency and in some cases specifically for selection and discrimination (in a current example from Germany, they are used by people who want to change their energy supplier). In addition, there is a justified need to experiment and drive development forward – how can we build trust and not gamble it away?
Finn: By pointing out potential, sharing success stories and assessing the ratio of unobjectionable to problematic applications. Industrial applications, for example, do not use data from private individuals and they help to make work better and safer. For every application we discussed, there are four that have tacitly changed things for the better – from curing diseases to mitigating environmental disasters.
“In the use of today’s algorithmic applications, we still face many questions, both technically and socially”
Lajla: When using today’s algorithmic applications, we still face many technical and social questions, e.g. about human-technology interaction. Therefore, we have to take a close look at particularly sensitive areas (personnel selection, health and public services). Certification in these areas could create more security for users and those affected.
Where do you see a particularly urgent need for action in European legislation? Do you find the approach of exclusively risk-related regulation sensible? How could the EU as a legislator perhaps even send a positive signal now?
Finn: With its exclusive focus on the most conscientious regulation possible, the EU will find that it is difficult to retrospectively impose their own standards on foreign companies that drive innovation – as was the case with the tech giants of the 2000s. The local AI ecosystem must be backed much more rigorously: by public partnerships and funds, investments in education and professorships, the opening of test fields and the clarification of ambiguous regulation. The so-called ecosystem of excellence is notoriously under-emphasised.
Lajla: With the GDPR, Europe has shown that it can play a pioneering role in tech regulation issues. Future AI regulation at European level can add another chapter to this success story if it creates binding standards for applications. This can also offer small and medium-sized companies or start-ups a secure framework for innovations. Risk-related regulation combines innovation promotion and necessary standards. Nevertheless, trustworthy AI not only requires laws but also supervisory institutions and contact points for citizens.
Good solutions require both the provision of and access to (personal) data to a greater extent than before. As a society, we need to be more understanding and willing to disclose this data in the future. Do you agree with this statement?
Finn: With a self-critical glance at our social media use, I fail to see a lack of willingness to share data. And while there certainly is a trend towards mass data collection, not all of it is profitable. It will increasingly be a matter of awareness: where is my data? What data do I never want to reveal? With regard to questions of transparency and liability, I envision a strong role for the regulator. And governments have started to pick this up.
Lajla: Agreed, Finn! Already today there are zettabytes of data lying around unused on servers. But who owns them? Large foreign tech companies. I would like citizens and civil society to harness the potential of the data for themselves. This primarily requires intelligent data-sharing models and more examples of how data can be used for joint projects, for example, through projects like the Gieß den Kiez tree-watering project by CityLAB Berlin.
This interview was first published in our annual research magazine, encore.
This post represents the view of the author and does not necessarily represent the view of the institute itself. For more information about the topics of these articles and associated research projects, please contact info@hiig.de.
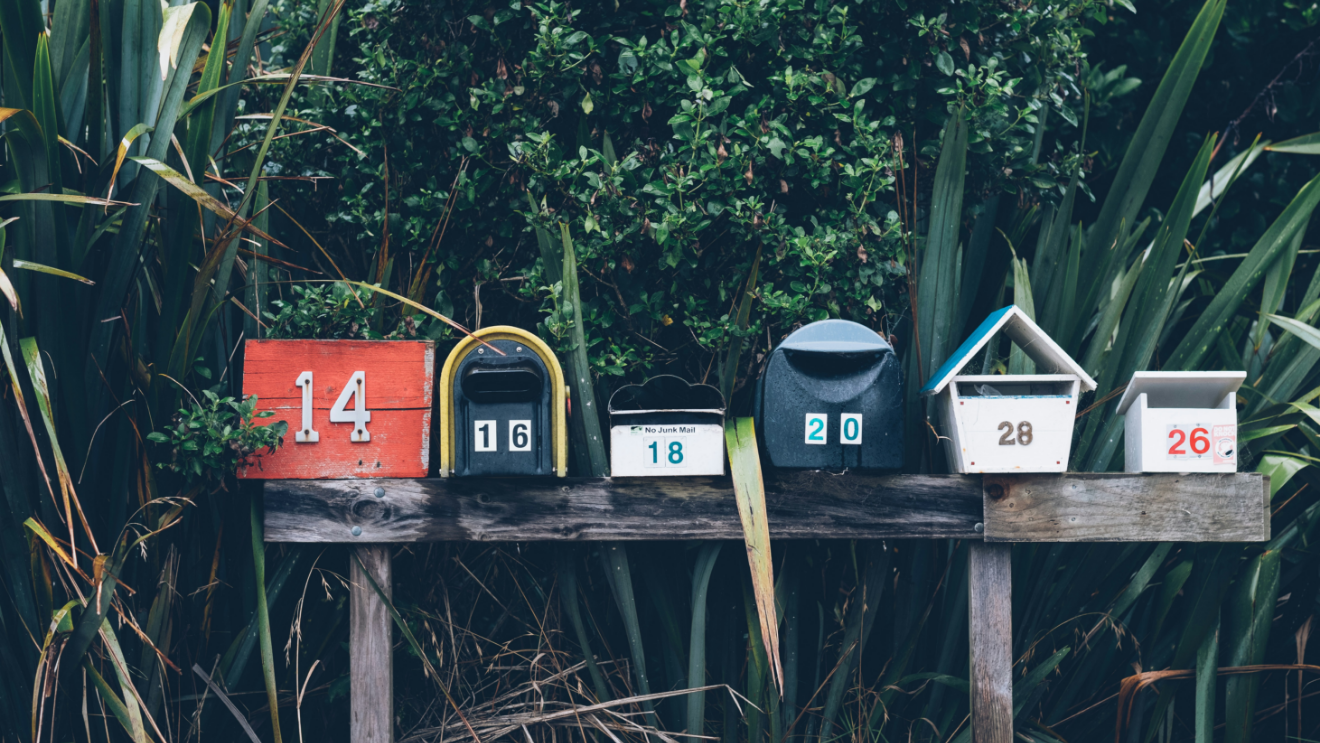
You will receive our latest blog articles once a month in a newsletter.
Artificial intelligence and society
Escaping the digitalisation backlog: data governance puts cities and municipalities in the digital fast lane
The Data Governance Guide empowers cities to develop data-driven services that serve citizens effectively.
Online echoes: the Tagesschau in Einfacher Sprache
How is the Tagesschau in Einfacher Sprache perceived? This analysis of Reddit comments reveals how the new simplified format news is discussed online.
Opportunities to combat loneliness: How care facilities are connecting neighborhoods
Can digital tools help combat loneliness in old age? Care facilities are rethinking their role as inclusive, connected places in the community.