Making sense of our connected world
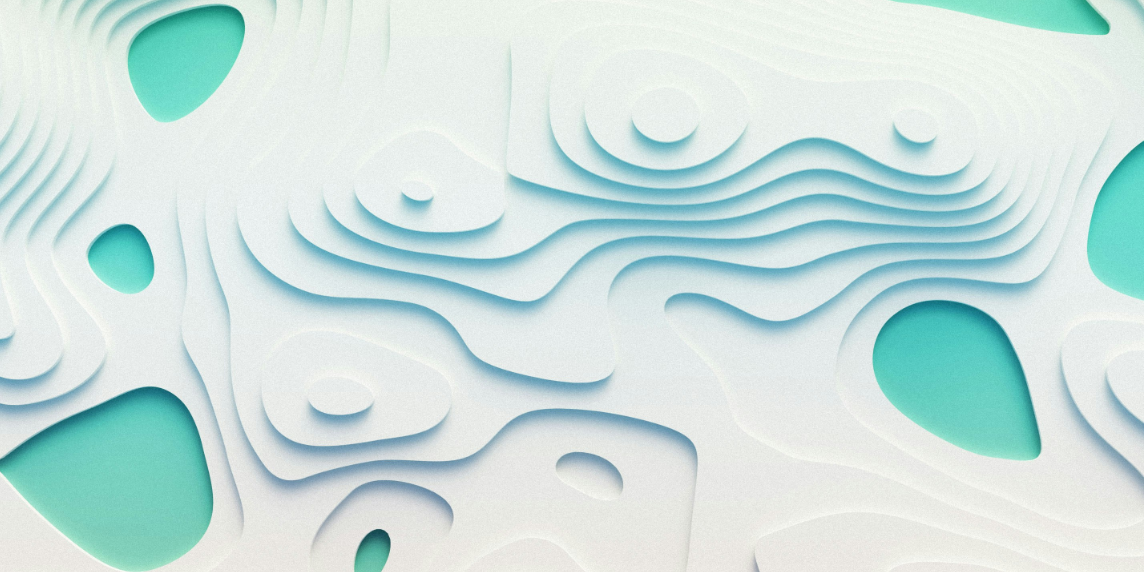
Artificial intelligence with purpose: Mapping the landscape of public interest AI
How can artificial intelligence (AI) be harnessed meaningfully for the public good? The research group Public Interest AI has developed a dataset documenting AI projects that prioritise social value over profit. The dataset comprises a wide range of initiatives in fields such as environmental protection, healthcare, education, social justice and democratic participation. It provides researchers and society with valuable insights into the practical application of AI for the common good. This article outlines how the dataset was developed, what information it contains, and how it can support a better understanding and development of public interest AI projects. But how can the long-term impact of these projects be measured, and how can their concrete social value be assessed?
What is public interest AI?
Defining public interest AI is challenging, as the concept of the common good varies depending on social and political contexts. In our Public Interest AI project, we understand the common good, following Barry Bozeman, as “serving the long-term survival and well-being of a collective understood as the public” (Bozeman, 2007). In this sense, the development of AI applications is not about private or commercial interests such as profit maximisation, but about benefiting the community as a whole. While applications like ChatGPT or DALL-E are primarily designed to provide services to individuals or businesses, public interest AI projects deliberately use their technologies to address societal challenges. AI-powered diagnostic tools or intelligent environmental monitoring systems, for example, aim to enhance equal access to information or tackle climate change, thereby creating a positive impact.
One example is the EU-funded SEACLEAR project, which is developing a solution to automate the collection of waste from the seabed. The project employs various technologies, including robotics and AI, to identify underwater areas where rubbish is located.
Another public interest AI tool is Simba, developed at HIIG, which promotes equitable access to information. This freely accessible, open-source and non-commercial application reduces language barriers online. It includes an internet app to simplify user-generated text and a browser extension that automatically summarises and simplifies web content. Simba’s goal is to make complex language more understandable, especially for people with learning difficulties or limited German proficiency.
Other public interest AI projects may operate as non-profit organisations. However, our research shows that profit motives often clash with, or at least create tension with, the goals of the common good. Further theoretical considerations on the notion of the “public good” and its connection to AI have been discussed by research group lead Theresa Züger and Hadi Asghari from HIIG in this article. An overview of key principles can also be found on the project website.
Why is the data we’ve collected important?
The use of AI for social purposes—often referred to as “AI for Good,” “AI for the public interest,” or “AI for social good”—is gaining traction and increasing attention in public discourse. Major players like Google, Microsoft, and public institutions are investing in public interest AI: Google awarded AI for Social Good grants in 2021, the German Environment Ministry funded sustainable AI projects through its “AI Lighthouses” programme, and Microsoft runs its own AI for Good Lab.
Despite these initiatives, there has so far been no detailed and structured overview of public interest AI projects. Such an overview is essential to understand the current landscape, evaluate the actual impact and potential of these initiatives, and identify remaining gaps. Without structured documentation, it’s unclear which societal issues are being addressed with AI, what technologies are being used, and where further action is needed. So how can we improve visibility and networking among these initiatives?
Filling the Gaps
To address these gaps in practice, our Public Interest AI team developed a comprehensive dataset between 2022 and the end of 2024. It provides open access to detailed information about the projects. This allows us to track developments over time, identify success factors, and facilitate connections among stakeholders. The dataset also enables informed political and academic decisions to support and advance public interest AI in a targeted way.
A comprehensive dataset is the foundation for exchanging knowledge about the evolution, challenges, and opportunities in the use of AI for the common good. With this knowledge, a sustainable landscape of public interest AI projects can be better understood and supported. The provided dataset is intended to offer researchers as well as policy actors in this field improved oversight and detailed access to data. We also hope that the dataset will help identify new public interest AI initiatives and thereby expand the field.
Which AI projects are included – and why?
The dataset includes applications aimed at social benefit rather than private or commercial gain. Each project either develops or applies AI in the public interest. It is important to note that the dataset is not a representative sample, but it is the most comprehensive collection of public interest AI projects known to us.
Projects are documented using 14 structured attributes, some categorised and others recorded in free text. Categories include project location, type of AI technology used, profit orientation (for-profit or non-profit), responsible institution, and project status.
A key feature is alignment with the United Nations Sustainable Development Goals (SDGs). Each project is reviewed by two people and matched to at least one relevant SDG. If it contributes to multiple goals, all are documented accordingly. The 2030 Agenda, with its 17 goals, aims to combat global poverty, protect the planet and promote prosperity. Specific targets include health and well-being, quality education, gender equality, climate action, and peace and justice.
As mentioned earlier, SEACLEAR contributes to SDG 14: Life Below Water, specifically to Target 14.1, which addresses marine pollution reduction. Simba also aligns with an SDG: Goal 10, Reduced Inequalities. Complex language can be an invisible barrier. By translating texts into plain language, Simba improves access to information, contributing to Target 10.3, which promotes equal opportunities and reduces discrimination.
Another dataset category identifies the type of AI technology used, including Computer Vision, Machine Learning (ML), and Natural Language Processing (NLP). For instance, SEACLEAR uses Computer Vision to locate marine debris, while Simba uses NLP for automatic text simplification. Where projects provided this information directly, it was used; otherwise, classification was based on project descriptions and functionalities. Hadi Asghari’s technical expertise was invaluable in this process.
Additional attributes include funding sources, project location, responsible institution, website link, and a brief project summary. A detailed description of all features can be found in the dataset’s ReadMe file. Some gaps remain, as not all necessary information is publicly available for every project.
How was the data collected?
Two methodological approaches were used to collect the data:
Network-based method
The first approach involved network-based identification (labelled as “network search” in the dataset). This method drew on the research group’s professional networks and targeted searches to identify relevant projects. Each project was checked against our minimum definition of public interest AI and documented using the dataset attributes. In the absence of existing compilations, this proved to be the most effective and reliable method for systematic documentation.
Structured web-based method
The second approach involved a structured web search using a predefined set of keywords in an online search engine. The top 20 results were reviewed, and links on those pages were followed up to two levels deep. Every page within this depth was scanned for relevant projects to include in the dataset. To minimise personalisation bias, searches were conducted in incognito mode with cookies rejected and no logged-in accounts. However, search results may still vary depending on the user’s device and location, which could not be fully controlled, making complete reproducibility impossible.
What comes next?
The dataset is publicly available under a CC-BY licence on Hugging Face and continues to grow. Projects that develop or apply AI in the public interest can be suggested externally or submitted directly by project teams.
Being included in the dataset offers multiple benefits: increased visibility within a global network of public interest AI actors, opportunities to connect with similar initiatives, and greater chances of being noticed by researchers, funders or policymakers. Projects that complete the detailed questionnaire appear on the global Public Interest AI Map, a central platform showcasing public interest AI initiatives.
The more complete and diverse the dataset becomes, the better we can understand the field, identify opportunities, and tackle challenges. This dataset is a first step towards making the landscape of socially beneficial AI technologies more visible.
References
Bozeman, B. (2007). Public values and public interest counterbalancing economic individualism. Georgetown University Press.
This post represents the view of the author and does not necessarily represent the view of the institute itself. For more information about the topics of these articles and associated research projects, please contact info@hiig.de.
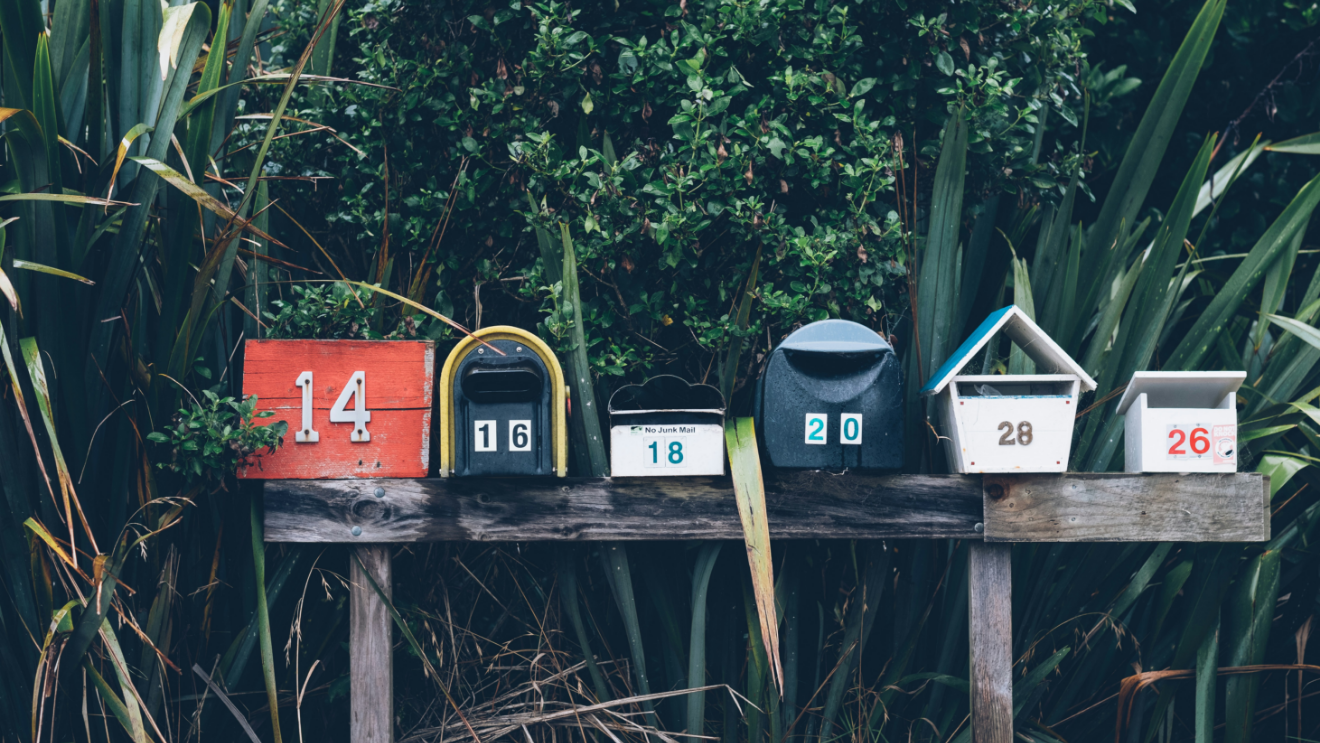
You will receive our latest blog articles once a month in a newsletter.
Digitalisation and sustainability
Artificial intelligence and society
Opportunities to combat loneliness: How care facilities are connecting neighborhoods
Can digital tools help combat loneliness in old age? Care facilities are rethinking their role as inclusive, connected places in the community.
Unwillingly naked: How deepfake pornography intensifies sexualised violence against women
Deepfake pornography uses AI to create fake nude images without consent, primarily targeting women. Learn how it amplifies inequality and what must change.
Who hired this bot? On the ambivalence of using generative AI in recruiting
Generative AI in recruiting promises efficiency, but may also quietly undermine the human connection that HR decisions and candidate fit rely on.