Making sense of our connected world
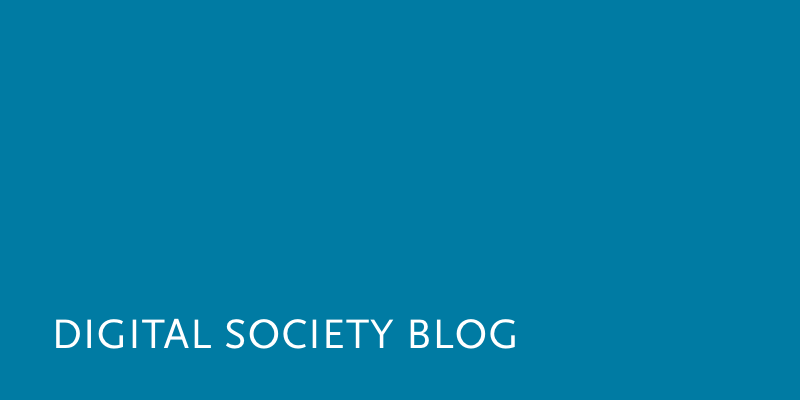
Data sharing: Why it’s useful, why still nobody does it and where it could lead to
According to a study from Tenopir et al. (2011) among 1300 US scientist, 67 per cent of all respondents considered the missing access to research data as a major drawback for scientific progress. In the same study, only 36 per cent of the interviewed scientists said, they would openly share their research data with others. In a naive assessment of the study: Although the most researchers recognize the importance of data sharing, only a few really share.
Why data sharing is useful
The advantages of openly available data are obvious: Through open data, other scientists can easily reproduce a study and thereby review and assess its results. Openly available data can make ad hoc reliability and objectivity analysis possible. Other scientists could even hit upon new insights in old data. Synergy effects wherever you look – the quintessence is clear: Sharing makes science more efficient.
In addition, the same argumentation mantra that accompanies the Open Access movement holds true for data sharing: Data, which have been collected from tax money, should be available for everyone. Everyone should have the right to access research data. Basta!
Why scientists still don’t do it
Interestingly do both, the efficiancy and the financing argument explain an individual action (data sharing) with a societal benefit. A certain public service obligation is thereby always ascribed to the action of a scientists. An assumption that does not fully reflect the research practice. In the ‘real life’, scientists experience a couple of impediments for data sharing, how several studies show.
Data is not yet currency
Haeussler et al. (2011), in an experimental study, emulated a classical prisoner dilemma for scientists. A scientists had to decide whether he or she shares a partly solution to a problem with other scientists. An interesting result of the study was that the more value a scientist would assign to his or her insight, the lower is the probability he or she would share it. Transferred to data: The more profit a scientists expects from his data (e.g. publication), the more likely he or she would withold the information.
This examples reveals an actual problem: The researchers’ profit is mostly linked to the publication not to the underlying data. Data only reveals its true value after its narrative processing; even though data analysts could probably use a clean dataset much more than the text. To share data depends on the individual calculation of its value; it has socioeconomic dimension. Scientists do not share because they often have nothing from it. Instead, sharing data – even after the publication – is laborious. One has to clean the dataset, find an appropriate format, enrich it with meta data and upload it. The costs of sharing are high.
Missing standards
There is an absence of clear conventions for data sharing within many disciplines; it lacks clearly formulated information on which metadata needs to be listed and how data needs to be formatted. This is why there are rarely applicable software tools and repositories – a problem that also Nelson (2009) mentions. Disciplines like math and physics do already use data archives like arXiv.org; other disciplines however lack conventional repositories and quality standards. It shows that data sharing has also a infrastructural and policy dimension: Where can data be stored? How can it be found? And who sets the standards?
Sure, these arguments do not yet cover the entirety of possible data sharing impediments. There are for example also disciplinary pecularities like privacy issues in social science inquiries. Here sharing data has also an ethical or legal dimension. Another example is proprietary data – where sharing touches exclusive exploitation rights. One can also criticize the ceteris paribus tendency in my argumentation – a scientists is after all not a purely driven by self-interest. Still, I assume that individual participation barriers are a major starting point to establish a fertile data sharing culture.
4 columny of a data sharing culture
Incentives: Scientists only share their data if they get a certain profit from doing so (see Haeussler’s study). Thus it needs a gratification structure for sharing data, for instance impact metrics for sharing that take into account the popularity or effort of a dataset (here).
Data standards: It needs clear disciplinary data conventions that state the quality standards and metadata structure. The question that occurs is: Who sets these standards? Politics? Infrastructure provider? Journals? Private companies?
Keep the effort low: In connection with datastandards, the effort of processing of data to a generally useable format needs to be as low as possible. No scientists would spend more time with the processing of data than with its evaluation.
Findability: Sharing data does not end with making it available. Open available data needs to be tracked easily, for example through search engines. It needs data brokers for the mediation of research data. Again the question occurs: Who could do that?
Does data sharing lead to ‘factory science’?
Looking at the demands mentioned above, one can recognize a certain tendency of decoupling the product from the producer. Who says that those who collect data are also responsible for its exploitation? The typical data lifecycle in fact allows for a multitude of possible specializations: Collecting data, managing and maintaining data, analyzing data and publish articles. All of these are actually just steps in a modularized process; a process that could possibly as well be handled by a multitude of specialists instead of one generalists. And mass computing and ICTs are rather fostering factors. Science has in this regard striking parallels with a car production; and everyone knows that there is not enough room on the radiator bonnet of Mercedes for everyone who helped producing it.
This post is part of a weekly series of articles by doctoral canditates of the Alexander von Humboldt Institute for Internet and Society. It does not necessarily represent the view of the Institute itself. For more information about the topics of these articles and asssociated research projects, please contact presse|a|hiig.de.
This post represents the view of the author and does not necessarily represent the view of the institute itself. For more information about the topics of these articles and associated research projects, please contact info@hiig.de.
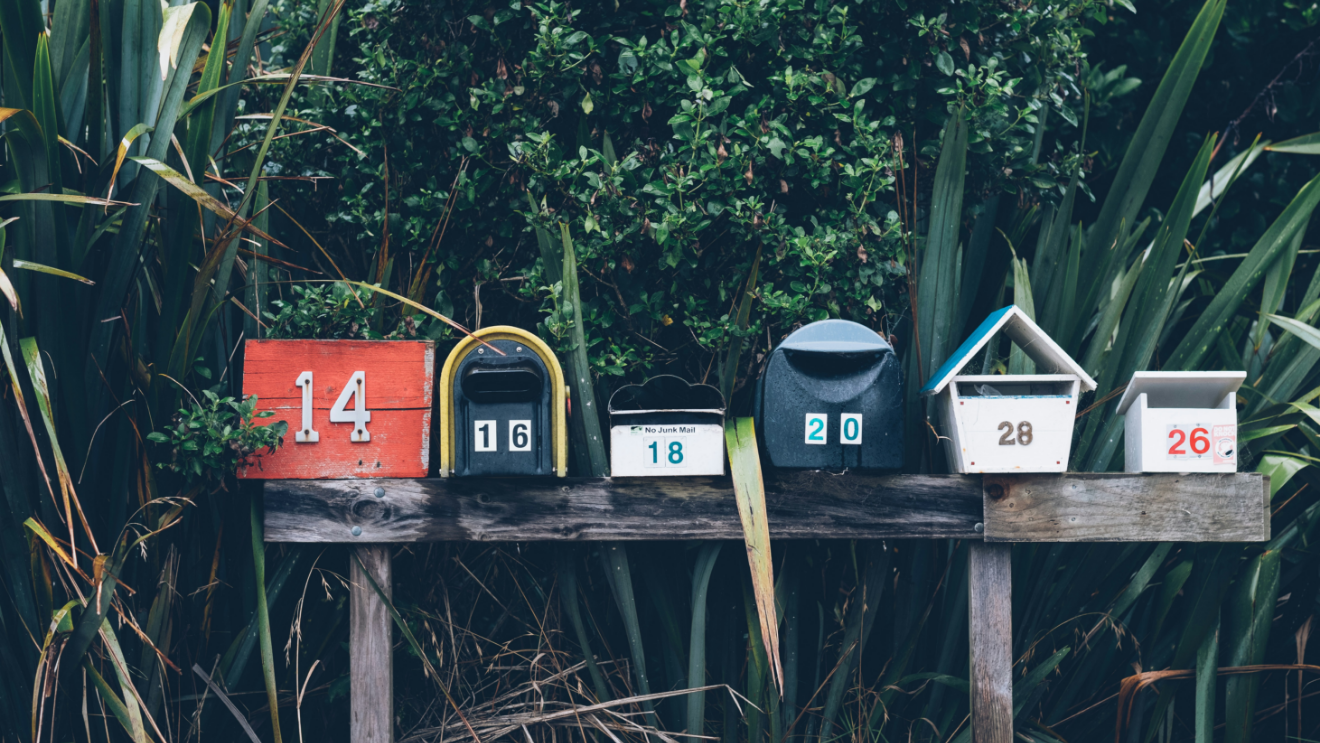
You will receive our latest blog articles once a month in a newsletter.
Research issues in focus
Online echoes: the Tagesschau in Einfacher Sprache
How is the Tagesschau in Einfacher Sprache perceived? This analysis of Reddit comments reveals how the new simplified format news is discussed online.
Opportunities to combat loneliness: How care facilities are connecting neighborhoods
Can digital tools help combat loneliness in old age? Care facilities are rethinking their role as inclusive, connected places in the community.
Unwillingly naked: How deepfake pornography intensifies sexualised violence against women
Deepfake pornography uses AI to create fake nude images without consent, primarily targeting women. Learn how it amplifies inequality and what must change.