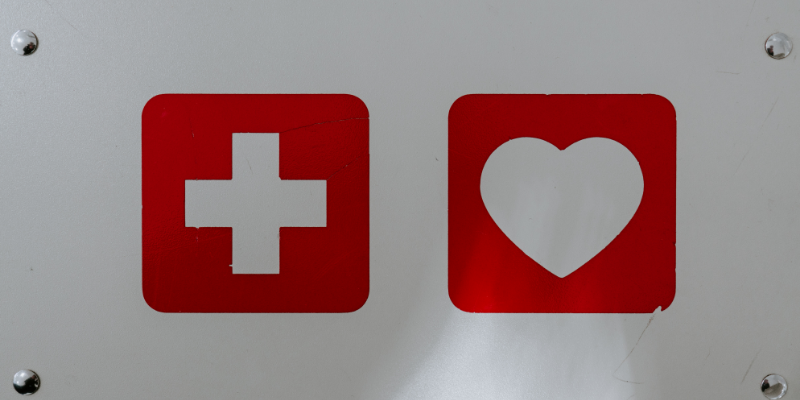
Human-centered data governance in health and care sectors
Even if actors are bound by common values and goals, they may have different ideas, interests and risk perceptions regarding the handling of data worth protecting. These ideas need to be brought together and the conflicting interests reconciled. This requires good data governance. We have developed a cooperative and inclusive data governance process that is pragmatic, goal-oriented and at the same time scalable.
Introduction
We have founded the Digital Urban Center for Aging and Health (DUCAH) as a human-centred research centre that conducts learning labs for transdisciplinary perspectives on digital health and ageing. Our vision is that our lives, health and ageing in a digital society will become more individual, more contextual, more data-based, more connected, more preventive, more dignified and at the same time more affordable. We want this to be possible through smart health systems that put people first and develop healthy neighbourhoods. This can be achieved with digital and social innovations – together with many research disciplines, in cooperation between the state, business and civil society. In order to contribute to this, we will generate, collect and process data within the framework of DUCAH. This also includes health data requiring special protection according to current data protection law.
Even though, at DUCAH, we are driven by common values and goals i.g. human centred promotion of health, we are well aware that we have many different and sometimes conflicting interests when it comes to dealing with data worth protecting. The DUCAH partners are shaped by their respective goals, methods, processes and structures, their different mental models and terminologies, but also their different perceptions of value and risk associated with the processing and use of data. And value and risks can change over time, depending on how the data is actually used.
To reconcile interests in data, its sharing and use, which diverge between the different actors involved in terms of the value of the data and the risks associated with its processing, we need appropriate data governance structures and processes. This requires, in particular, considering the contextuality of the value and risks of data as well as the influence of law on whether data is also legally fit for use. Further, we need to coordinate at the normative, organisational and technological levels and achieve an appropriate level of centralisation or decentralisation, standardisation and participation. We thus need a governance process that is scalable to negotiate small and simple, i.e. low-risk, as well as large and high-risk processing activities. Data governance must be flexible, if common understanding changes in the course of the negotiation, if a division into several processing activities allows for more differentiated solutions, or if apparent “side issues” arise or stakeholders express fears or concerns. It is about decidedly non-technocratic approaches to digitisation projects to create outcomes that are acceptable to all stakeholders. And that is why it is important to also clarify the “why” – why do we do this but not that, why do we choose this option over others – instead of relying on a command style.
A pragmatic process model for data governance
We have chosen a process-oriented approach because we start from the realisation that there is no universal, “one size fits all” solution. Instead, an appropriate approach needs to take into account the concrete constellation of actors, the context, the goals, interests, concerns and wishes of the stakeholders, the concrete data and the technical systems. We have therefore created a process over the past year. It develops, designs and implements a solution for a specific use case that balances all interests, meets all legal requirements, mitigates all risks and achieves the goals of all stakeholders. It is an iterative process that allows flexibility, brings together expert input and stakeholder inclusion (co-evaluation, co-creation & co-design), and has a strong solution orientation.
The six-step process model
The following six steps outline both, a typical generalised project process flow and the iteration part, which includes the identification of data, interests and derivation of data access, use and output and expresses the iterative nature of our model.
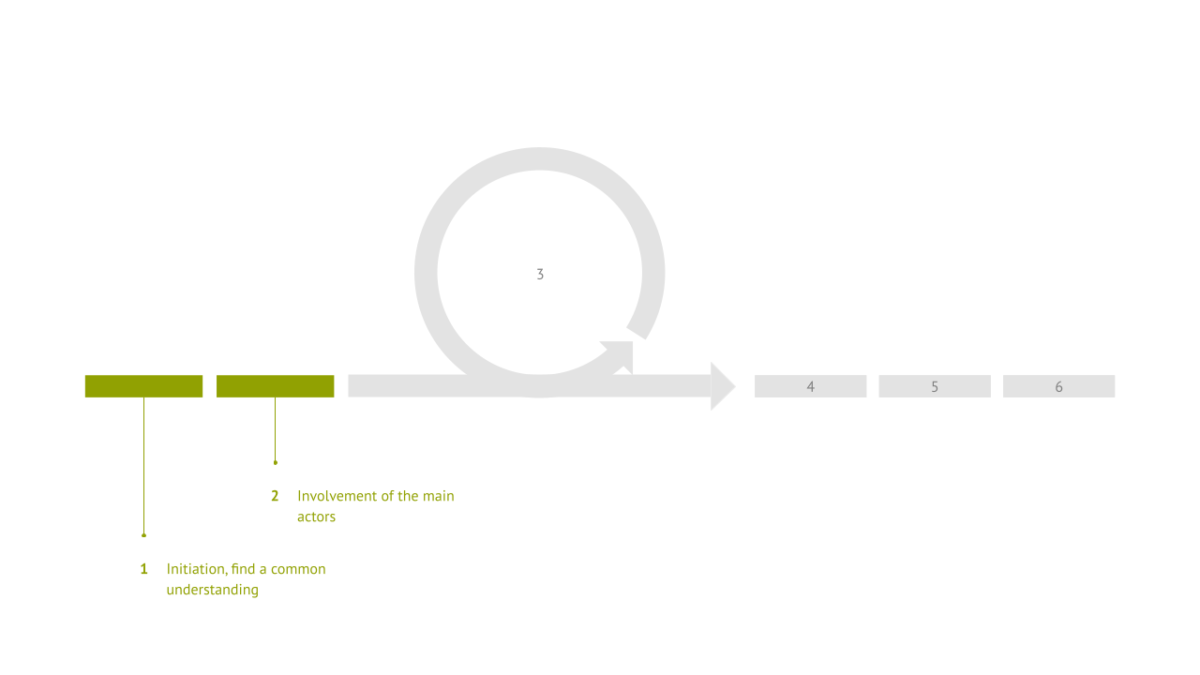
The first step is to bring together all the initiating stakeholders and find a common understanding of the project being pursued together. At this point, the partners may want to set up a cooperation agreement, and they may have to file ethics applications.
The second step is to identify and include all other necessary stakeholders. It must be clarified which stakeholders need to be involved directly and which indirectly, for example through representatives, and how appropriate formats of inclusion must be designed for the different stakeholders. In order to enable fair, meaningful and result-oriented inclusion, the formats should neither belittle nor overburden the stakeholders, be they care workers, people being cared for or patients.
The third step is the most central and serves the incremental participatory finding of solutions, which addresses all three dimensions we’ve identified as necessary – the normative, the organisational and the technical dimension as well as their interplay in combined legal-organisational-technical solutions. The aim of this step is to jointly create a consented processing design that meets the interests, needs and expectations of all stakeholders. The third step comprises the inner loop, which gives process-oriented data governance the necessary adaptability.
The inner loop: a path to iterative solution finding
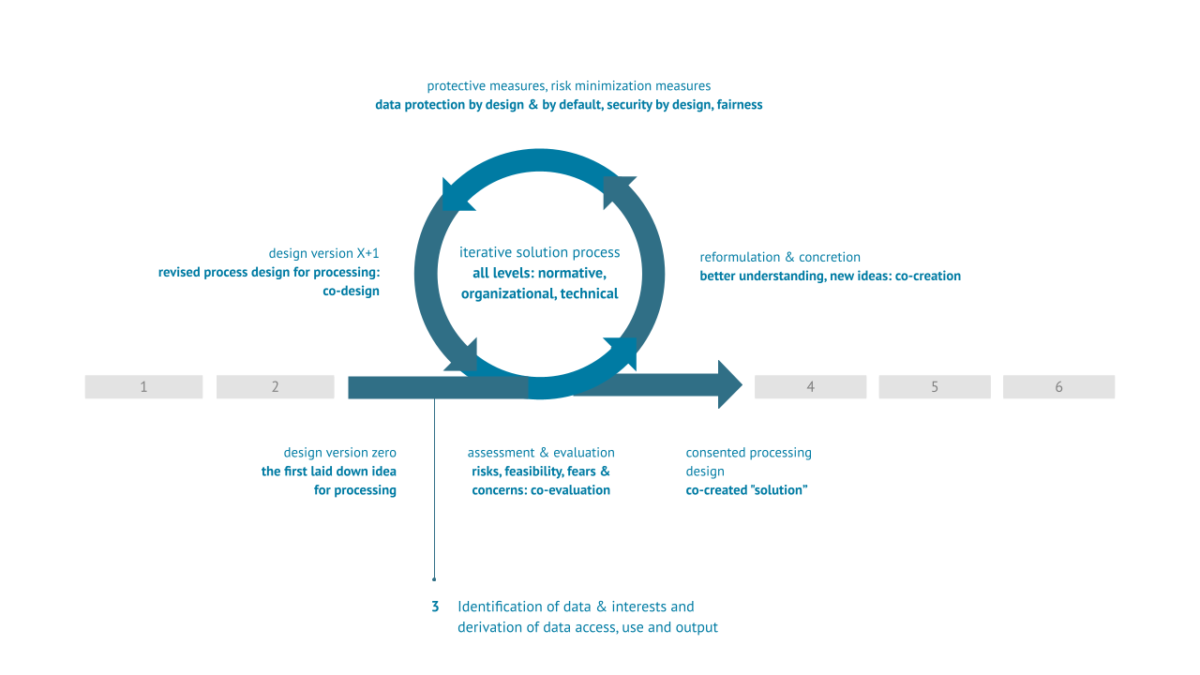
The iterative process starts by developing an initial processing design, perhaps just a first rough idea, and by clarifying what data exists or is to be collected, what goals are to be achieved or insights gained, and what technical systems are to be used to achieve them.
This initial processing design has then to be assessed and evaluated: In terms of the conditions that need to be met or created, the feasibility of the processing, in technical as well as in practical terms, the implications and risks it might entail, the expectations, fears and concerns of the stakeholders. This assessment and evaluation require an inclusive approach, bringing all stakeholders to the table, for a truly co-evaluation endeavour.
Depending on the outcome of the participatory assessment and evaluation, the iterative process either ends with a consented processing design – if all interests and expectations are met – or one needs an additional iteration: reformulation & concretising, protective measures, processing design revision, and then again a participatory assessment and evaluation.
The reformulation and concretisation step is strongly influenced by co-creation formats and should allow stakeholders to take a new look at the processing, to gain a better understanding of it and to generate new ideas. Often it turns out that the stakeholders can achieve the same goals by taking different paths or thinking outside the box, and in the process solve many riddles, concerns and risks the previous processing design generated. An important finding from practical application is that many problems can be solved by splitting a more complex and more challenging processing design into several, much less complex processing designs. These are each easier to understand, less challenging, less risky and less prone to generate concerns.
Integrating special expertise
The next step in the iterative process, the incorporation of protective measures, is, then, to draw on expertise from different fields and disciplines to incorporate measures into the processing design to ensure compliance with all applicable laws, the protection of all stakeholders and their rights and interests, and to minimise risks and undesired implications of the processing. This expertise should cover topics such as information and cyber security, data protection (by design and by default) and other areas of law, as well as transparency, fairness and explainability in AI and algorithms.
Based on the reformulation and concretisation as well as the incorporation of protective measures, the processing design is to be revised and redesigned. This stage again requires an inclusive, participatory approach with adequate co-design formats.
This revised processing design has then again to be assessed and evaluated – and depending on the outcome, the iterative process may start again. Apart from a very few high-risk processing operations that require multiple iterations of this iterative process, we know from both the research literature and practical experience that rarely more than two iterations are required before the processing design meets the interests, needs and expectations of all stakeholders.
The remaining steps of the pragmatic data governance process model
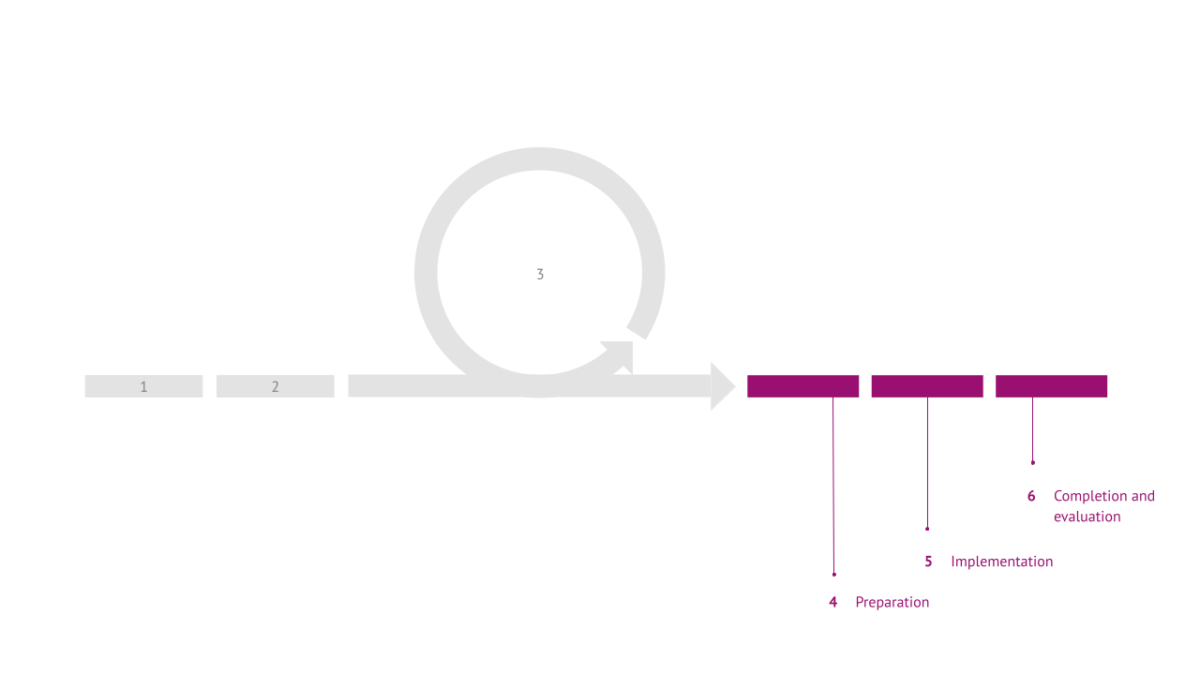
The fourth step builds on the consented processing design and aims at preparing the successful implementation of the project. This includes preparing appropriate information material, drawing up contracts, for example with subcontractors, and declarations of informed consent, perhaps also preparing and submitting joint applications for third-party funding, but in any case jointly agreeing on work plans and schedules. When everything is ready, the project can start.
The data governance process continues and accompanies the project in the implementation and execution phase. The fifth step includes, among other things, possible introductory or accompanying workshops for the various stakeholders. But above all, it is about ensuring that data collection, processing and use are accompanied and that questions and challenges that arise are addressed in good time before they can become problems.
Shared learning for future projects
After the completion of the project, the sixth and final step is the completion of the data governance process, evaluation and shared learning for future projects and accompanying data governance. This includes, for example, final workshops for the stakeholders, the scientific and practical exploitation of the project results, and the deletion or anonymisation of the collected data. The shared learning from implementing the data governance process is tremendously important, not only because it allows the process itself to evolve, but also because it empowers all stakeholders to tackle larger and potentially riskier projects together in the future. They, thus, gain confidence in themselves and trust in the other stakeholders that together they can exploit the value of the data and achieve results without their interests, needs and expectations being violated.
Closing remarks
We’ve already successfully tested the data governance process model in practical use cases in the health and care sector. For example, in a facility for residential care for the elderly in Zehdenick in Brandenburg. In this facility, we set up a measuring device to collect indoor ergonomic data and addressed the data governance issues with the process defined above. Some of the most central aspects of the process model could thus already be validated, to the great benefit of the stakeholders. These include the openness to address even seemingly minor aspects and concerns openly, collegially and productively, because they may nevertheless be considered very important by individual stakeholders. It also includes the possibility of dividing complex processing, for which the stakeholders cannot find an acceptable solution, into several simpler processes and solving each of them individually. Last but not least, we have shown that the process allows legal requirements, for example from the EU General Data Protection Regulation, to be implemented in a verifiable manner.
We continue to work on the further development of the process model, above all, to improve its practical applicability and to enable stakeholders to carry out such data governance processes themselves. To this end, we work on guiding questions and success criteria for the individual process steps as well as on the development and integration of concrete formats for successful co-evaluation, co-creation and co-design. Next year, the authors plan to bring all of this together and provide a practical manual with concrete recommendations for action.
References
Jackson, P., Schildhauer, T., Ulich, A., Pohle, J., & Jansen, S. A. (2021). Aging, independent living and technology. Stiftung für Internet und Gesellschaft. DOI: 10.5281/zenodo.5026032 More Information
Grafenstein, M. v., & Ulich, A. (2021). Data-Governance-Framework für das Digital Urban Center for Aging and Health (DUCAH). Stiftung für Internet und Gesellschaft. More Information
Schildhauer, T., Ulich, A., Winckler, P. (2021). Tech & aging: How to enable independent living with digital innovations. encore, 2021/2022, 160-167. More Information
Ulich, A. (2021). Wie wird das digitale Gesundheitssystem fit für die Menschen? Gesundheit, Alter und Data Literacy. In: Renz, A., Etsiwah, B., & Burgueño Hopf, A. T. (eds.), Whitepaper Datenkompetenz. Berlin: Universität der Künste, pp. 27–29. More information
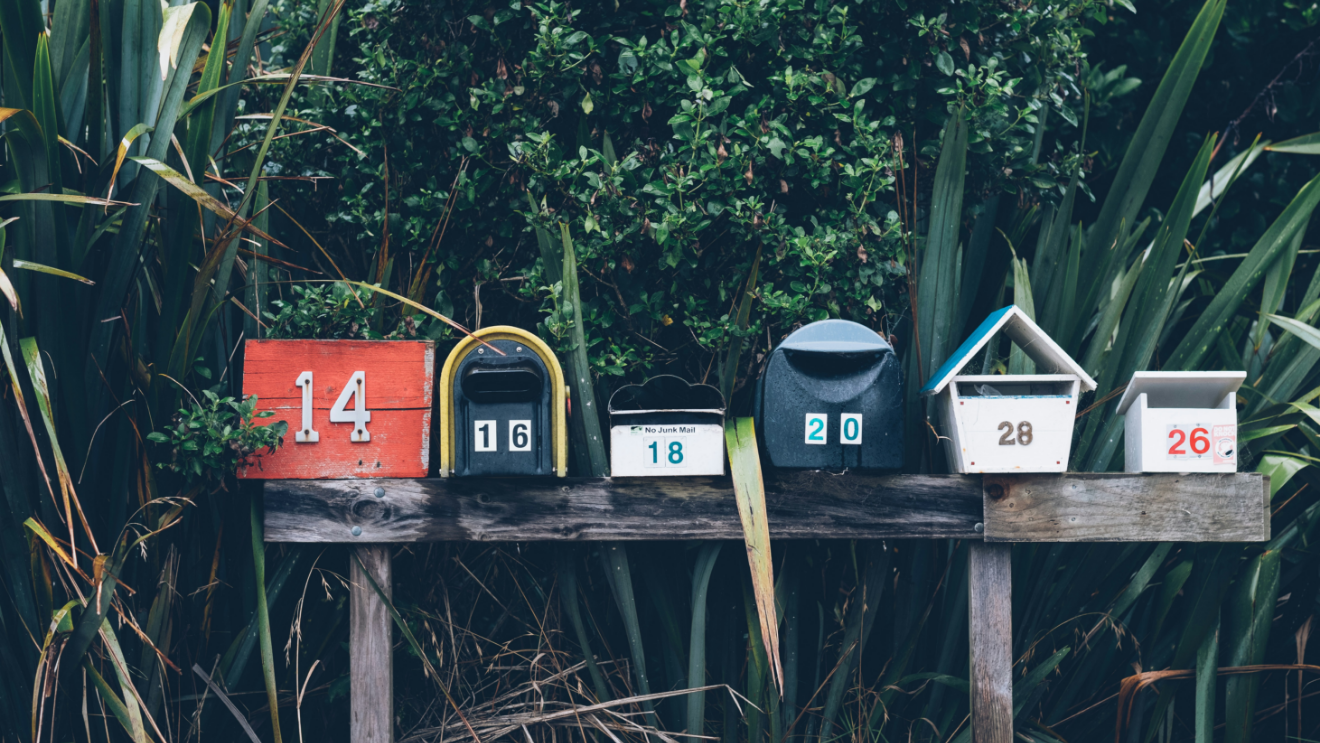
You will receive our latest blog articles once a month in a newsletter.
Data governance
Escaping the digitalisation backlog: data governance puts cities and municipalities in the digital fast lane
The Data Governance Guide empowers cities to develop data-driven services that serve citizens effectively.
Online echoes: the Tagesschau in Einfacher Sprache
How is the Tagesschau in Einfacher Sprache perceived? This analysis of Reddit comments reveals how the new simplified format news is discussed online.
Opportunities to combat loneliness: How care facilities are connecting neighborhoods
Can digital tools help combat loneliness in old age? Care facilities are rethinking their role as inclusive, connected places in the community.