Making sense of our connected world
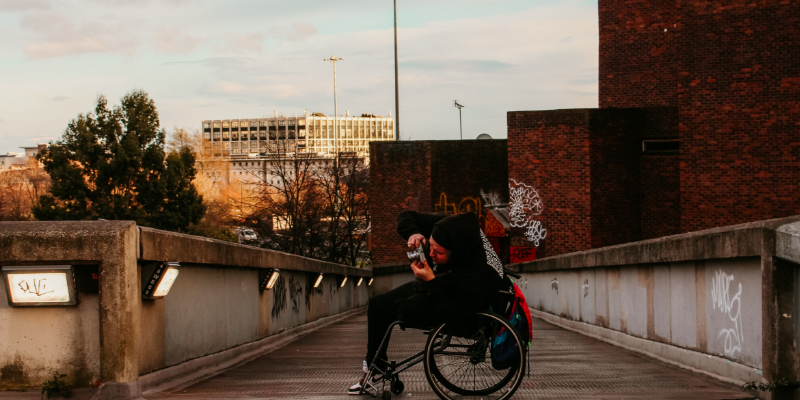
Exploiting potentials: Teaching AI Systems to See Accessibility Barriers
Despite explicit political support for the removal of barriers in our built environment, they are still widespread. Therefore, AI-supported mapping and documentation of barrier-free accessibility holds a lot of potential for people who are limited in their mobility. On the one hand, it provides them with the necessary information to navigate through their everyday life in public space. On the other hand, by making problems visible, it creates general incentives for effective improvement measures. But how exactly can AI support us in this task? The first step is to provide open training data. We present such a dataset for detection of steps and stairs.
Introduction
The United Nation’s convention on the rights of persons with disabilities, which entitles everyone equal access in the physical environment and to transportation, has been signed by all EU member states. But the actual state of accessibility is still far from reaching these standards. Progression is slowly being made, but for many, dealing with barriers is still part of everyday life.
This problem has inspired grass root initiatives such as Wheelmap.org. The platform collects information on wheelchair accessibility of public spaces worldwide and integrates this information with Open Street Map. This makes it possible to look up the accessibility of places, given that they have been mapped. Everyone can contribute to the project by entering the status of unmapped places, thus expanding the available knowledge bit by bit.
Similarly relying on the help of digital volunteers, the Project Sidewalk from the University of Washington maps high curbs, lowered curbs and sidewalk obstacles from Google Street View imagery. This makes it easier to plan A to B wheelchair navigation, a task that is currently difficult due to lacking or misleading information in current mapping options.
Projects like the above have an immediate benefit of providing people with better knowledge to make informed planning decisions. Additionally, such citizen generated data has potential to push and guide public governance, thereby supporting the agenda of improving accessibility in the built environment. Unfortunately, the data collection by the volunteers is an immense task, which could greatly benefit from some degree of automation. This is where AI comes into the picture.
AI for accessibility mapping?
Modern computer vision technology can efficiently extract information from image data. Project Sidewalk has already successfully integrated automatic object detection techniques in combination with voluntary annotations to improve efficiency and accuracy. Other ideas in this regard include classifying surface types of public paths from image data.
Applying AI technologies to such tasks inherently depend on training the systems on collected data. When creating such training data it is important to respect the individuality of accessibility issues and the complexities this involves. This is best done by not trying to achieve AI systems aimed at inferring the accessibility of a place directly, but rather tools that can detect relevant information.
Project Sidewalk greatly exemplifies this approach: Rather than inferring whether sidewalks are accessible, they use object detection techniques to recognize relevant objects such as curbs, lowered curbs and sidewalk obstacles. Inferring accessibility can then be left as a combination of knowledge of such features, the precision of the models and individual or specific use case requirements, eventually leading to more versatile utility of the technology.
Data for step and stair detection
Another critical hindrance to wheelchair accessibility of public spaces, as also emphasised in the Wheelmap mapping guide, is steps or stairs at the entrance to a place. As a first step in facilitating automatic detection of such barriers, we have created an open dataset of 5538 images with 3564 steps, 1492 stairs and 143 wheelchair ramps annotated. The photos are all provided by Wheelmap volunteers and depict places that have been mapped on the Wheelmap platform, which also means a majority of the images are from Germany.
The principles for annotation have been decided in collaboration with Prof. Dr. Benjamin Tannert, who is an expert in technology for accessibility. The data can be found here, where we also provide more detailed descriptions of how the data was annotated and discuss its possible flaws and biases.
We hope this open dataset can prove valuable in the endeavour of documenting barriers in the built environment. Furthermore we hope it will provide a useful input in the discussion of sensitive data design and transparent annotation practices. It is important to acknowledge that data is not objective. Even when focusing on object level annotation, non-trivial choices will always be made, which eventually determines what falls into which categories, what will be detected and on which types of data input the model will work.
Often these choices arise from a tension between the data needing to be simple and heterogeneous for machine learning algorithms to work well and at the same time trying to encompass the diversity of real world situations. Compromises in this regard will always be made, which is why transparency on such choices and the consequential biases is important in order to facilitate correct usage of the data later. In this regard, we are also very interested in feedback and ideas for improvement.
This post represents the view of the author and does not necessarily represent the view of the institute itself. For more information about the topics of these articles and associated research projects, please contact info@hiig.de.
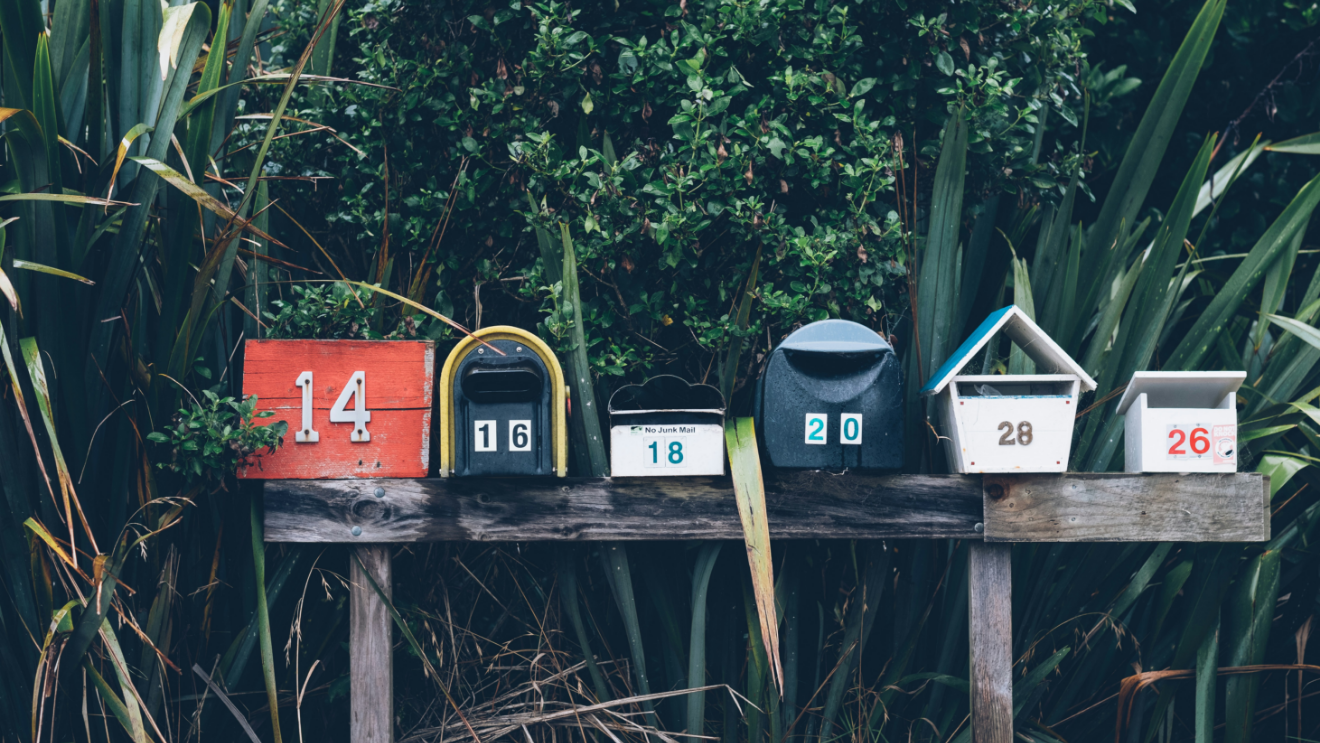
You will receive our latest blog articles once a month in a newsletter.
Artificial intelligence and society
Navigating platform power: from European elections to the regulatory future
Looking back at the European elections in June 2024, this blog post takes stock of the Digital Services Act’s effect in terms of navigating platform power.
AI Under Supervision: Do We Need ‘Humans in the Loop’ in Automation Processes?
Automated decisions have advantages but are not always flawless. Some suggest a Human in the Loop as a solution. But does it guarantee better outcomes?
The plurality of digital B2B platforms
This blog post dives into the diversity of digital business-to-business platforms, categorising them by governance styles and strategic aims.