Unsere vernetzte Welt verstehen
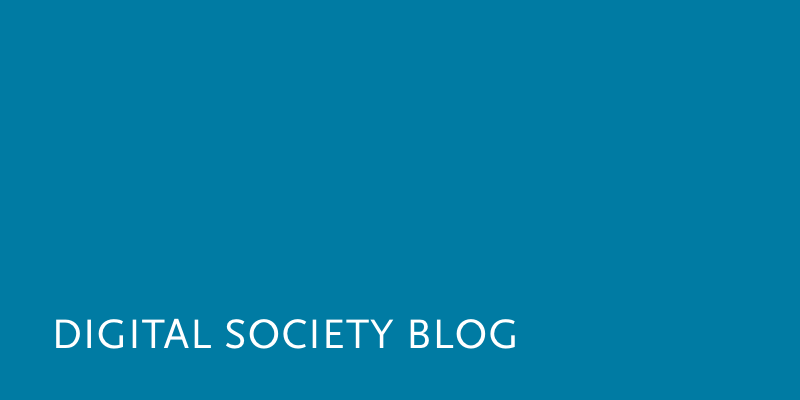
Mehr datengetriebene Beschleunigung!
Die Entrepreneurship-Forschung hat bisher wenige für Unternehmer ausreichend anwendungsorientierte Publikationen produziert. Praxisorientierte Veröffentlichungen wie bspw. “The Lean Startup” von Eric Ries kompensieren das und geben Unternehmern verständlichere und einfache Vorgaben bzw. Entscheidungsregeln. Da Unternehmen in der Regel sehr unterschiedlich sind, müssen auch diese Regeln mit Erfahrung angereichert werden. Ein relativ neue, institutionalisierte Form dieses Erfahrungsaustausch sind Accelerator-Programme. Als relativ neues Phänomen in der Startup-Landschaft, haben diese Programme über die letzten Jahre ein enormes Wachstum und Popularität erfahren. Eine transparentere und ausgeklügeltere Datensammlung und -austausch könnten nicht nur das Startup-Ökosystem mit interessanten Erkenntnissen zum Prozess von Startups bereichern, sondern auch neue, anwendungsorientiertere Forschungswege aufzeigen.
This blog post is a call for accelerator programs to be more transparent regarding their performance and more rigorous in gathering data on the progress of their startups. Along with research, this can have a significant positive impact on the eco-system as a whole.
With the amazing upsurge of such programs over the last years, the benefits of this valuable data to research and practice are of extreme value. While a comprehensive list is hard to find (and maintain), f6s lists 799 such programs as of Jan 22, 2016 (http://www.f6s.com). Techstars (http://www.techstars.com), being one of the most well-respected and successful accelerators, lists >20 accelerator programs within its own organisation. In the following, the necessity, benefits and a first proposal towards a more data-driven acceleration process is described.
Shortcomings of entrepreneurship research
Entrepreneurship research has not been very practical in giving startups recommendations actionable enough to be useful. While seminal publications such as Shane’s “The Individual-Opportunity Nexus” present interesting research concepts, they hardly provide any operationalizable guidelines for (to-be) entrepreneurs.
The problem has been partially offset by a stream of practically oriented handbooks such as Steve Blank’s “Customer Development” framework and Eric Ries’ “The Lean Startup”. These books have been written by practitioners for practitioners and provide clear guidelines and recommendations on what to do.
The entrepreneurial thirst for such publications has been massive indicating the need for more actionable recommendations as opposed to sophisticated but only theoretical concepts. As useful these books have been for entrepreneurs to alleviate the practicality issue of entrepreneurship literature, they are qualitative in nature and thus leave (a lot of) room for interpretation.
This is a natural problem since startups can be very different in many areas such as team composition, capabilities, product offering, resources etc. Recommendations therefore always represent a tradeoff.
Yet, the problem of more quantifiable and data-driven recommendations persists. Having a more quantified decision basis can help founders calibrate their activities along questions such as “Am I doing the right things?” and “Am I doing the right things in the right way (e.g., fast enough)?”
Experts necessary to overcome this
To find the right answers to these questions, startups leverage experts and mentors or make use of trial-and-error. These practitioners are able to give a calibration based on their specific expertise and experience with a wide range of situations. They can fit the general framework to the specific startup’s situation.
A recent more institutionalized way to supply startups with the necessary “calibration” for their activities are accelerator programs. Such programs typically supply cohorts of startups with different services such as mentoring and networking along with a limited amount of money over a period of a few months. The basic idea is to make the startups investor-ready and enable them to raise the necessary funding after the program. The basic assumption behind these programs is that things can happen faster when adding the right level of mentorship and advice (along with a network at ). Over the course of these programs, startups are pushed to their limits and are typically expected to achieve more than they would without the program. The acceleration is not only based on longer working hours, but on skipping unnecessary activities and doing the right activities as fast as possible.
During my research, I got to know different accelerator programs and have interviewed both startups as well as accelerator managers to better understand what is going on. One recurring theme and source of benefit for startups is the calibration aspect: Founders are able to calibrate what they do and how they do it both with their peers within the program as well as with experienced mentors. They are especially able to calibrate the time necessary for specific activities, i.e., the speed at which they progress. By doing this, they can achieve specific milestones in a faster fashion by skipping unnecessary activities and spending less time on “over-polishing” other activities. Also, they are able to observe within the cohort the achievements of other startups and are thus more motivated.
Accelerators (potentially) at the forefront of entrepreneurship research
With speed being a major expectation among investors and stakeholders nowadays, being able to calibrate their speed can be the difference between success and failure for startups. They are expected to build scalable business models fast, to deliver a proof-of-concept early on to enter into the cycle of funding. All that requires them to do the right things in the right way. Given their limited resources, over-delivery can be a risk.
What is currently missing, is a broader dataset of what can be achieved by early-stage startup by when. Given the data-driven everything nature of entrepreneurship, this is a pity. Startups oftentimes specifically need experts to understand what is achievable within a week, a month or a year instead of leveraging the lessons learned from thousands of other startups. Many of them waste scarce resources on unnecessary activities or spend too much time on a task – potentially overcomplicating them without coming closer to their goals.
Measuring startup progress – not quite easy
Since startups – especially in their early phases – rarely have meaningful data on more traditional success metrics such as revenue or profit, progress needs to be measured differently. One alternative would be to use more operational metrics, which are easily measurable and are very likely to occur as milestones during the early phases of a startup. Milestones are binary and have either been hit or not – as simple as measuring something can get. Examples for such binary metrics include the following:
- Discovered opportunity
- Specified idea
- Performed interviews with prospects
- Defined minimum viable product (MVP)
- Finished MVP
- Performed first sale
- Attracted first external client
- Attracted first accelerator-induced client (e.g., corporate stakeholder)
- Produced first pitch
- Received external funding
- Generated first revenue
- Achieved break-even
- Achieved profitability
While a list like this creates the instant impression of linearity in a process known as the opposite of this, it is a necessary first step. These measures explicitly or implicitly occur in the different frameworks out there and are oftentimes part of the feedback startups are given by mentors. Given a large enough data base, patterns will emerge to reduce the complexity of the innumerable amount of potential combinations of milestone occurrences (even increased by the iterative nature of the startup process). Advice can then be substantiated in data.
These binary metrics refer to the existence of something rather than its quantity. As a startup matures, it will most likely hit all of these milestones. Measuring its progress will therefore shift to other more traditional measures where measuring the development over time actually makes sense:
- Revenues
- #Clients
- Size
- Profitability
Major opportunity to step-change entrepreneurship (research)
Currently, accelerator programs do not abound in published data. While they (sometimes) publish information on the startups they accept or the ones that receive follow-on funding, a more operational level of quantification is missing. Sources such as Seed DB are a good starting point and offer some data, which is very descriptive and not suitable to base recommendations or conduct research on it. Current numbers indicate that accelerators are helpful for startups to increase the probability of follow-on funding. The longer term impact on the sustainability of the accelerated businesses is still to be proven.
On the other hand, accelerators offer very fertile ground to better understand what is happening over the course of the entrepreneurial process for early stage startups. Despite the fact that there has been entrepreneurship research in entrepreneurship over the last decades, many gaps still exist. Part of the reason has been the fast evolvement of the entrepreneurial process over recent years. Technological developments have enabled startups to be faster and more iterative. Accelerators have the potential to enable research to catch up with this. They offer many benefits to function as an appropriate environment to observe startups and learn:
- They have a steady flow of startups being exposed to knowledgeable mentors, i.e., a lot of data can be gathered relatively fast.
- Despite many differences, they offer a certain level of comparability, e.g., regarding the stages of a startup.
- They offer different specializations and can therefore be useful to identify differences between different sectors, business models etc.
The beneficiaries of a more open approach to data gathering and sharing would be:
- Accelerators: With the number of accelerators ever growing, publishing data to quantify the acceleration could (and should) be a major competitive advantage to accelerators.
- Startups/Ecosystem: Startups, which don’t participate in such programs can profit from the learnings, potentially be more successful and therefore enrich the eco-system (which is beneficial for all).
- Research: Additionally, generating data over time would enable researchers to identify patterns and develop more actionable recommendations for startups.
Dieser Beitrag ist Teil der regelmäßig erscheinenden Blogartikel der Doktoranden des Alexander von Humboldt Institut für Internet und Gesellschaft. Er spiegelt weder notwendigerweise noch ausschließlich die Meinung des Institutes wieder. Für mehr Informationen zu den Inhalten dieser Beiträge und den assoziierten Forschungsprojekten kontaktieren Sie bitte info@hiig.de.
Literature
- Shane, S., 2003. A General Theory of Entrepreneurship: The Individual-opportunity Nexus. Edward Elgar Publishing.
- Ries, E., 2011. The lean startup: how today’s entrepreneurs use continuous innovation to create radically successful businesses. Crown Business, New York.
- Cohen, S., 2013. What Do Accelerators Do? Insights from Incubators and Angels. innovations 8, 19–25.
- Isabelle, D.A., 2013. Key Factors Affecting a Technology Entrepreneur’s Choice of Incubator or Accelerator. Technol. Innov. Manag. Rev.
- Clarysse, Bart, Mike Wright and Jonas Van Hove, 2015, A look inside accelerators – Building businesses (NESTA)
Dieser Beitrag spiegelt die Meinung der Autorinnen und Autoren und weder notwendigerweise noch ausschließlich die Meinung des Institutes wider. Für mehr Informationen zu den Inhalten dieser Beiträge und den assoziierten Forschungsprojekten kontaktieren Sie bitte info@hiig.de
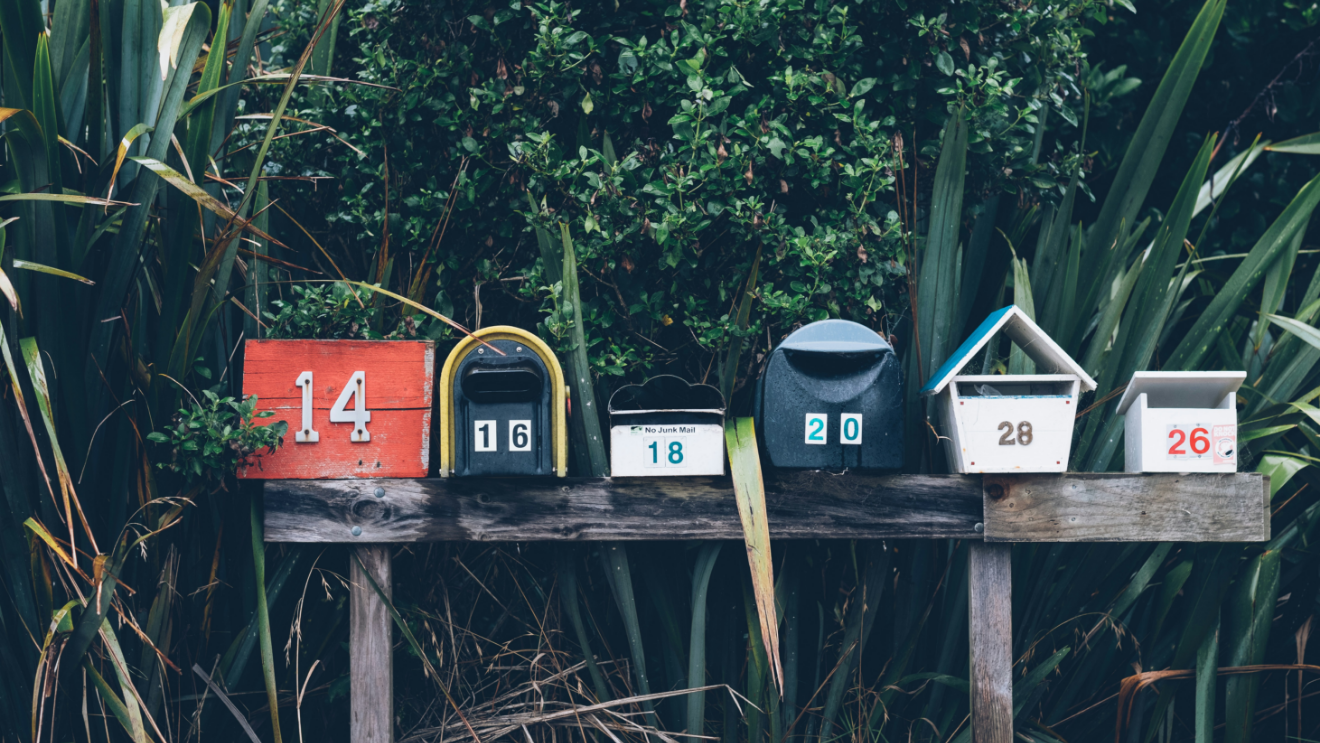
Jetzt anmelden und die neuesten Blogartikel einmal im Monat per Newsletter erhalten.
Plattform Governance
Netzecho: Reaktionen auf die Tagesschau in Einfacher Sprache
Seit 2024 gibt es die Tagesschau in Einfacher Sprache. Wie wird das neue Nachrichtenformat von Nutzer*innen im Internet diskutiert?
Chancen gegen Einsamkeit: Wie Pflegeeinrichtungen das Quartier vernetzen
Was hilft gegen Einsamkeit im Alter? Pflegeeinrichtungen schaffen neue Räume für Gemeinschaft und digitale Teilhabe.
Unfreiwillig nackt: Wie Deepfake Porn sexualisierte Gewalt gegen Frauen verschärft
Deepfake Porn nutzt KI, um täuschend echte Nacktbilder ohne Einwilligung zu erzeugen, meist von Frauen. Wie können wir Betroffene besser schützen?